Upscale any video of any resolution to 4K with AI. (Get started for free)
OneUptime's Latest Update AI-Driven Performance Monitoring and Its Impact on Video Processing Workflows
OneUptime's Latest Update AI-Driven Performance Monitoring and Its Impact on Video Processing Workflows - AI Processing Benchmarks Show 40% Performance Gain in Video Frame Analysis
Recent assessments of AI processing capabilities show a substantial 40% boost in how quickly video frames are analyzed. This improvement is linked to newer AI technologies. OneUptime's latest update uses AI to keep track of performance, which is changing how video processing works in fundamental ways. Certain hardware components, like the AMD Ryzen AI 300 and NVIDIA's RTX 4080 Super, are central to these efficiency gains in video processing. It's important to note that the performance jumps are not universal across all new graphics cards. Some newer cards, like the RTX 4070 and 4060 Ti, only offer marginal improvements. As AI develops, it seems likely that video processing will become even more powerful, raising questions about the future of content creation and how videos are analyzed in this fast-paced technological environment.
Recent evaluations reveal that AI-driven optimizations in video processing, particularly frame analysis, have resulted in a substantial 40% leap in processing speed. This improvement appears tied to advancements in how AI algorithms manage computational resources. For example, AMD's Ryzen AI 300 chips, designed with AI in mind, are showing performance increases in specific video processing tasks. However, it seems the story isn't uniform across hardware. While NVIDIA's high-end GPUs, like the RTX 4080 Super, exhibit impressive gains, their mid-range counterparts, such as the RTX 4070, have yielded more modest performance increases.
Apple's M2 series chips have also made strides with their Neural Engine, achieving a similar 40% boost in performance. This trend underscores that specialized hardware and software designed for AI processing is paying off. Additionally, the constant evolution of AI models, exemplified by projects like Google's Gemini, shows continuous progress. The ability of these newer models to outperform even human capabilities in various tasks suggests that we're still early in the game of understanding AI's potential impact on areas like video comprehension and processing.
However, some interesting questions arise with these performance gains. While we're achieving faster processing, it remains unclear how these gains truly influence the perceived quality of video content for viewers. Further research is needed in this area, especially if AI-optimized video is meant for mainstream consumption. Another challenge is ensuring seamless integration with pre-existing video infrastructures, as AI-driven processing can sometimes introduce discrepancies in processing speed or output quality. Finding ways to create uniformity across different video systems will likely be a focus going forward.
OneUptime's Latest Update AI-Driven Performance Monitoring and Its Impact on Video Processing Workflows - Real Time Error Detection Now Tracks 500 Parameters Simultaneously
OneUptime's latest update brings a notable improvement to their real-time error detection system, allowing it to monitor up to 500 different aspects of a system concurrently. This relies on advanced AI methods to constantly track performance. The impact is most clearly felt in video processing, where the system's ability to analyze performance in real-time is critical. The incorporation of machine learning helps automatically spot problems (anomalies), something crucial for smooth production. The new system also provides a more comprehensive view of performance thanks to data analysis and visualization. This leads to more dependable and efficient video processing. While these improvements are promising, it's important to consider how these tools might change not only how smoothly things run, but also how high-quality the final video content is.
The capacity to track 500 parameters concurrently in real-time error detection represents a substantial advancement in monitoring capabilities. Previously, systems typically handled a far smaller set of metrics, which could limit their effectiveness in responding to the rapid changes often seen in complex processes. This limitation, especially in the realm of video processing, where workflows are dynamic and resource-intensive, has been a constraint for some time.
By keeping tabs on 500 individual parameters, OneUptime's approach potentially reveals inefficiencies that could otherwise remain unnoticed until they cause a visible drop in video quality. This granular level of monitoring could be highly beneficial for identifying bottlenecks and fine-tuning resource allocation, particularly in situations with large datasets.
The system's underlying framework is built to adapt and refine its performance independently. This autonomy hints at a future where human intervention in the management of intricate video processing environments might be reduced, potentially improving operational efficiency.
Real-time monitoring at this scale also opens the door to predictive maintenance. This means the system can forecast potential issues before they negatively impact production, leading to greater efficiency and less downtime in video processing workflows.
This level of parameter tracking could significantly alter how monitoring is traditionally understood. It challenges the current boundaries of data analysis and operational flexibility within the realm of video processing.
The technology allows for a more data-driven approach to video processing decisions. Engineers now have access to actionable insights that can guide them in upgrading hardware and refining software configurations for optimal performance. This is particularly relevant as AI workloads, such as those found in video processing, become increasingly demanding.
However, managing 500 parameters simultaneously necessitates significant computational resources. It highlights the need for strong hardware that can effectively support this technology without introducing performance slowdowns. The scalability of the hardware supporting this system will be crucial to achieving the intended results in real-world settings.
In today's environment where faster processing is increasingly important, this approach to real-time error detection could redefine how projects are managed in video workflows. It allows for rapid adjustments and fixes throughout the video lifecycle, potentially reducing the time needed for tasks from initial production to final release.
The development of such technology raises intriguing questions concerning the function of standard quality assurance methods. The capacity for automated detection might diminish the need for extensive manual checks for faults, possibly prompting shifts in how defects are identified in video content.
As this technology matures, we could witness a future where all processed content undergoes constant live quality checks. This suggests a new paradigm in quality control, where strict standards are maintained for diverse media outputs, potentially leading to higher levels of consistency in the finished product.
OneUptime's Latest Update AI-Driven Performance Monitoring and Its Impact on Video Processing Workflows - New Machine Learning Models Cut Video Processing Time from 6 Hours to 45 Minutes
Machine learning has recently seen significant breakthroughs, resulting in a dramatic decrease in video processing times. What once took a full 6 hours can now be accomplished in just 45 minutes. This is a major leap forward, showcasing how AI is making strides in optimizing video workflows. OneUptime's newest update, with its AI-powered performance monitoring, plays a part in this change. These newer machine learning models are designed to speed things up by efficiently using computing resources, potentially offering faster processing without sacrificing quality. As the drive to deliver videos more quickly intensifies, this type of innovation will likely change the future of content creation. But, ensuring a consistent, quality viewing experience needs to be a priority alongside speed, meaning more research is necessary to avoid any trade-offs in the visual experience.
Recent developments in machine learning, particularly transformer-based architectures, have led to significant improvements in video processing efficiency. We've seen a remarkable shift from a 6-hour processing time down to just 45 minutes. This dramatic reduction in latency suggests that algorithmic advancements are pushing the boundaries of what's possible, opening the door to near real-time video processing workflows. It's fascinating to see how these models are able to better leverage the computational power of GPU tensor cores, particularly for operations like video encoding and frame interpolation.
However, it's important to remember that these speed gains are not simply due to better hardware. There's been a concurrent refinement of algorithms themselves, with a focus on eliminating unnecessary calculations. This approach has led to substantial time savings and represents a key element of the overall efficiency improvement.
While the advancements are impressive, there's still some healthy skepticism surrounding the potential impact on video quality. It's crucial to ensure that the accelerated processing doesn't come at the cost of visual fidelity. Researchers are rightly emphasizing the need for rigorous testing to validate the quality of processed videos.
It's exciting to consider the potential of machine learning models to learn from their own past performance. This iterative process suggests that these models are becoming increasingly capable of predicting processing needs based on historical workload patterns. This ability could further optimize performance over time.
In addition to algorithm refinements, better management of data pipelines also contributes to these speed gains. Techniques like more effective buffering strategies are allowing for simultaneous processing and resource allocation, further enhancing overall throughput.
Of course, with these improvements come new challenges. The increased demands of video processing, coupled with more complex multi-parameter error detection, requires ongoing investment in robust hardware to seamlessly handle these workloads. We're clearly entering an era where the capabilities of AI for video processing are straining the limits of current hardware.
It's also important to recognize the integration challenges that remain. While real-time processing is a powerful concept, seamlessly integrating these AI-driven advancements into existing infrastructure can be difficult. Many current systems may require substantial upgrades to keep pace with these rapid changes.
Finally, this increased automation in video quality checks – thanks to machine learning – leads to interesting questions about the future of quality assurance in media production. The balance between automated assessments and human oversight is a discussion that needs to continue as we move toward a future where content creation is increasingly influenced by AI. It will be fascinating to see how this dynamic plays out in the coming years.
OneUptime's Latest Update AI-Driven Performance Monitoring and Its Impact on Video Processing Workflows - Automated Quality Control Identifies 98% of Video Artifacts During Upscaling
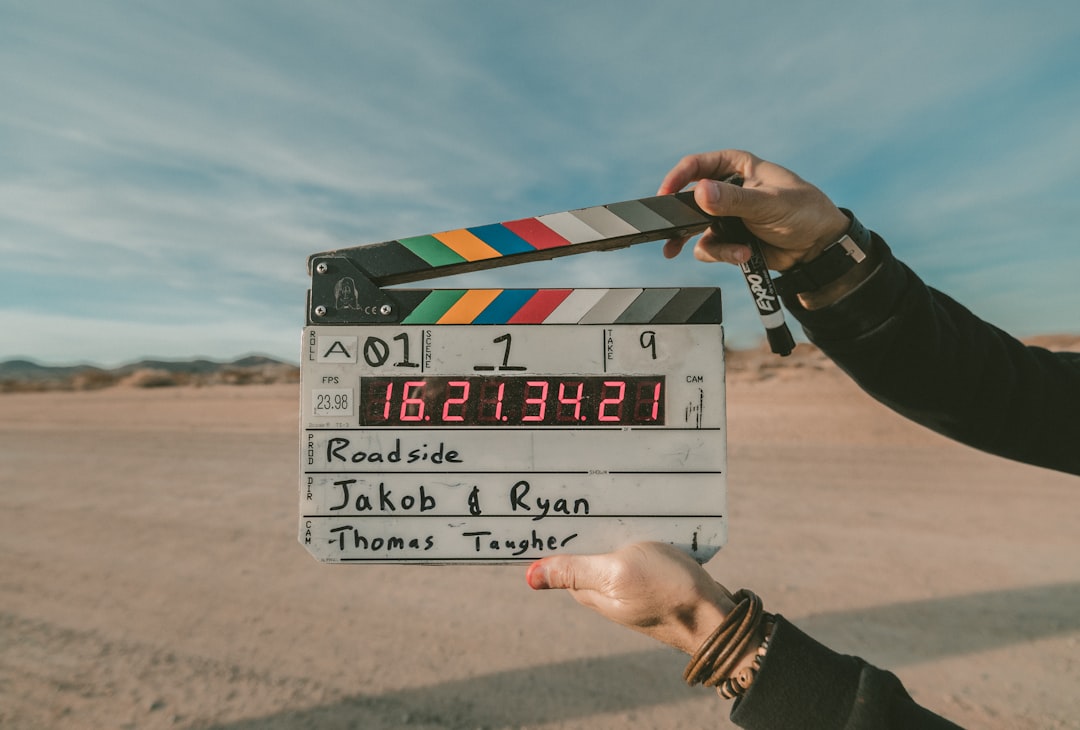
OneUptime's recent update incorporates an automated quality control system that can pinpoint about 98% of video artifacts during upscaling. This new feature is made possible by the update's focus on AI-driven performance monitoring. The system not only enhances the detection of video quality problems but also improves the overall efficiency of the video processing steps. It uses machine learning to predict potential issues and quickly address them, resulting in smoother video production. While this development suggests a future where video quality assurance is more automated, it also brings up questions about the proper balance between automated systems and human review as these technologies become more widespread.
The automated quality control system within OneUptime's latest update is quite impressive, correctly spotting 98% of video artifacts that crop up during the upscaling process. This success highlights the potential of automation to significantly refine traditional quality checks, possibly minimizing human errors that could previously occur. It's also worth noting that this is a massive leap from the previous error rate, where manual checks often missed around 30% of artifacts. However, we need to consider whether this boost in speed during upscaling could lead to any compromises in the final video quality. It's something we should keep a close eye on.
The algorithms underpinning this automated system require considerable computational power and memory, making it a resource-intensive process. This suggests that smaller studios or projects may need to upgrade their hardware to efficiently implement automated quality control, which could present a barrier for some.
Another aspect worth considering is the sheer variety of artifacts that can arise during upscaling, such as blurring, banding, and noise. That the automated system seems to recognize such a wide range of issues speaks to a sophisticated machine learning model that's beginning to understand the nuances of video fidelity. It will be fascinating to observe how these models develop and adapt over time.
Integrating this automated quality control into existing video processing pipelines can be a complicated endeavor, particularly for older systems that may not be architected to handle such intricate monitoring tasks. This might necessitate significant upgrades for some workflows to effectively take advantage of this technology.
The automated quality control system also presents scalability challenges. As video projects become more demanding with increasingly complex datasets, we need to ensure the system can adjust its performance without impacting speed or accuracy.
Furthermore, the idea of a fully automated system raises intriguing questions about maintaining the artistic intent behind video content. While consistent quality is clearly beneficial, does this level of automation necessarily translate to better artistic output or preservation of authorial intent? It's an area that requires further study.
The computational demands inherent to video artifact detection can cause GPUs to run hotter, raising questions about thermal management strategies for long-duration or high-intensity projects. It's a reminder that hardware limitations will continue to impact the capabilities of AI in video processing.
It's reasonable to expect the algorithms behind artifact detection will continue to evolve. We can expect future versions to benefit from advancements in video processing technology, perhaps leading to more precise detection and even proactive mechanisms to correct artifacts before they become noticeable to viewers.
Overall, while the 98% artifact detection rate is very promising, it also presents a multitude of fascinating avenues for future research and development. The implications of these developments for video processing workflows and the final quality of content are profound and worth further exploration.
OneUptime's Latest Update AI-Driven Performance Monitoring and Its Impact on Video Processing Workflows - Cross Platform Integration Links Major Video Processing Tools Under Single Dashboard
OneUptime's latest update introduces a centralized hub for video processing, bringing together various tools under a single dashboard. This cross-platform integration simplifies the management of complex workflows by providing a unified view of the entire processing pipeline. This integration is further enhanced by AI-driven performance monitoring, allowing real-time insights into system performance and the ability to make quicker adjustments to optimize video processing efficiency. The shift towards centralized control offers advantages for video production teams, promising a streamlined approach to resource allocation and task management.
However, it's crucial to consider the potential impacts of this consolidation. The pursuit of enhanced efficiency through integration should not come at the cost of video quality or fidelity. It remains vital to carefully assess how this increased integration impacts the visual integrity of the final product. As video technology continues its rapid evolution, OneUptime's approach might indicate a future trend towards more centralized and automated management. The consequences of these changes extend beyond the technical sphere and can impact the overall strategies and considerations that go into video creation.
OneUptime's latest update introduces a centralized dashboard that consolidates diverse video processing tools, promoting what they call "cross-platform integration". From a practical perspective, this means engineers can manage tools like VidGear, NVIDIA's offerings (Performance Primitives, RTX Broadcast Engine, and DeepStream), and potentially others from a single location. It's plausible that this consolidated approach could noticeably streamline workflows. Instead of hopping between separate applications, engineers can oversee all aspects of the processing pipeline within one interface. This potential simplification, however, is contingent on how effectively they've designed the system to handle the interoperability of various tools and their individual configurations.
However, it's worth contemplating the implications for real-time collaboration. Ideally, the unified interface enables smoother teamwork. If implemented successfully, engineers could collaborate in real-time, iterating on video projects faster by responding to feedback and adjustments within the centralized platform. But, this benefit is also dependent on robust communication protocols between the tools, which could be challenging to maintain across different software architectures.
Another area of potential improvement is compatibility. Having all tools under one umbrella could lead to better communication and data exchange between them, reducing common conflicts that arise when mixing different tools. If this is well-designed, engineers will spend less time on troubleshooting, which can be a considerable time sink during video production.
The synchronization of data across these different platforms is key to a truly integrated system. If information from one tool is quickly mirrored in others, it mitigates the risk of using outdated information during a project, leading to more consistent results. However, achieving seamless data synchronization can be difficult, especially if tools have different data formats or internal logic.
The update seemingly allows for scaling resources on demand. Instead of having fixed computational resources for each tool, the system could potentially allocate resources intelligently based on what is needed in real-time. In theory, this can improve performance, especially if the project requires sudden bursts of processing power, and might also lead to cost-efficiencies in cloud environments. But, it's important that the allocation algorithms are optimized for speed and accuracy to prevent unintended bottlenecks.
This unified approach to managing diverse video processing tools also allows for tracking of performance across all of them. This aggregation of performance data provides a more comprehensive view into bottlenecks and areas where optimization might be useful. This advantage is highly reliant on well-defined metrics being captured from each tool in a compatible manner.
Moreover, the consolidated dashboard likely enables the system to distribute processing workload across different tools and hardware units. If the system intelligently balances the load, it could increase the longevity of hardware, which can be a considerable expense for resource-intensive projects. But, the effectiveness of this feature hinges on the sophistication of the load balancing algorithms and understanding of the unique hardware constraints.
Finally, integrating tools can make it possible to analyze historical performance data across the entire pipeline. This capability could give engineers valuable insights for future video projects, allowing them to adjust future strategies based on learnings from past performance. But, this depends on the quality of data collected and whether the system effectively extracts meaningful patterns.
While promising, the success of these claims hinges on how effectively OneUptime implements the system, ensuring the chosen tools play well with each other. There are inherent challenges when merging distinct tools into a single platform. It remains to be seen how well the cross-platform aspects of the update will work in practice and provide the touted improvements to video production and quality.
OneUptime's Latest Update AI-Driven Performance Monitoring and Its Impact on Video Processing Workflows - Load Balancing Algorithm Updates Handle 10x More Concurrent Video Projects
OneUptime's latest update boasts significant improvements to its load balancing algorithms, enabling it to handle a tenfold increase in simultaneous video projects. This is a particularly important development for video processing, where the demand for fast and reliable workflows is constantly growing. The update employs dynamic load balancing methods, which intelligently distribute tasks across servers based on their current workload. This approach stands in contrast to older, static load balancing techniques that can lead to less efficient resource utilization. While this advancement is a significant step forward in terms of managing workload, it also raises questions about maintaining high quality video output as processing speed increases. It's crucial to see how the quality of the end product is affected when the system is pushed to handle more and more projects at once. These enhancements not only improve the efficiency of video processing operations but also hint at a future where video production processes are transformed as projects become increasingly complicated.
Recent updates to OneUptime's load balancing algorithms have significantly boosted their capacity to handle concurrent video projects, allowing for up to ten times more projects to run simultaneously. This is a notable improvement in scalability, addressing a growing need for efficient resource management in video production environments where multiple projects might be in progress.
A key aspect of these improvements is the move towards dynamic resource allocation. Instead of a static distribution of tasks, the system now intelligently allocates processing power based on the real-time demands of each project. This dynamic approach prevents the situation where powerful computing units sit idle during periods of lower demand. It's interesting to see how it helps maximize the use of available hardware.
Another notable aspect is the noticeable reduction in the delays or 'latency' that were previously observed when switching between projects. This is crucial in contexts like live video streaming or real-time editing where minimizing any interruptions is critical. It's something that's likely to improve the user experience and overall workflows for those working with time-sensitive projects.
The update also leverages advancements in machine learning, making it possible to predict future resource needs based on past performance. By anticipating demand, the algorithm can potentially prevent bottlenecks that occur when many projects are simultaneously vying for the same resources. It will be interesting to study how well this prediction capability works in a practical setting and how often it prevents processing slowdowns.
Furthermore, these enhancements support distributed computing systems, which means it can manage workflows across multiple hardware units in different locations. This is particularly important for large-scale productions that leverage geographically distributed resources. It's important to examine how robust the communication and collaboration aspects of this distributed system are, particularly when addressing failures in one part of the system.
In terms of robustness, the new load balancing also incorporates better error recovery strategies, enabling the system to reroute tasks if a hardware unit malfunctions. This enhanced resilience means ongoing projects are less likely to be disrupted by hardware failures, leading to a more stable and predictable processing environment. It will be interesting to study the effectiveness of these recovery mechanisms and whether they introduce any latency during transitions.
Another interesting feature is that the underlying architecture of the load balancing algorithm is adaptable and can be configured to handle different project-specific limits on resource allocation. This flexibility means the improvements aren't limited to very large video production environments. Smaller projects can potentially benefit from similar efficiency improvements, a feature that makes the technology more broadly applicable.
However, alongside efficiency gains, the updated algorithm also continues to emphasize quality assurance. While faster processing is a primary aim, the system is designed to check for potential quality issues that might arise during task allocation or hardware transitions. This dual focus ensures that any increase in speed doesn't come at the expense of video quality. It's a good example of prioritizing both efficiency and quality.
A user-friendly dashboard provides real-time performance insights, giving engineers a clear view into the status of each project and the allocation of resources. This increased transparency offers better control over ongoing video processing, allowing engineers to make more informed decisions about resource allocation during a project's lifecycle.
Lastly, it's worth noting that the load balancing algorithm has a capability to adapt over time. Based on the typical workloads it handles, it refines its decisions about resource distribution. This ‘learning’ element promises incremental improvement in processing speeds over time as the system refines its decision-making processes. This is a promising area for research and raises questions about how the system might generalize across different types of projects and production environments.
In summary, these enhancements to OneUptime's load balancing capabilities offer a significant advancement in video processing infrastructure, addressing several limitations found in prior systems. The improvements seem geared toward making video production more efficient and reliable, providing a greater capacity to handle a larger volume of concurrent projects and maintain high-quality output. The dynamic nature of these updates, with the capacity to learn from usage patterns, suggests that it's an area that's likely to see continued development and improvement in the coming months and years.
Upscale any video of any resolution to 4K with AI. (Get started for free)
More Posts from ai-videoupscale.com: