Upscale any video of any resolution to 4K with AI. (Get started for free)
The Impact of Free Downloadable Videos on AI Video Upscaling Techniques
The Impact of Free Downloadable Videos on AI Video Upscaling Techniques - AI algorithms analyze free video datasets to improve upscaling accuracy
AI algorithms are refining video upscaling techniques by analyzing readily available, free video datasets. These algorithms, powered by sophisticated machine learning methods, meticulously examine video footage, uncovering intricate details and textures previously missed by older, more rigid algorithms. The core of these AI upscalers lies in deep learning and neural networks, trained on these expansive datasets to produce higher-resolution outputs while minimizing artifacts and preserving crucial details. This shift signifies a growing reliance on freely accessible video content for training these AI systems, leading to improvements in upscaling accuracy. As these AI video upscaling tools continue to evolve, we can anticipate not only faster processing speeds but also a general increase in the quality of upscaled videos, thereby making high-quality video production more attainable for users of varying expertise.
AI algorithms are increasingly leveraging freely available video datasets to refine their upscaling capabilities. These datasets, often sourced from academic repositories or initiatives like Creative Commons, provide a vast pool of video frames that can be analyzed for patterns crucial to upscaling. These patterns help AI models intelligently fill in the missing details that are lost when videos are compressed to lower resolutions.
It's intriguing that even seemingly minor differences within video content can have a major impact on the effectiveness of AI training for upscaling. Datasets with diverse lighting conditions, motion types, and scene variations seem to be key to making the AI more adaptable. The AI can generalize better across a wider range of video types when presented with this sort of variety.
A common technique used with free video datasets is data augmentation. Essentially, the AI is presented with synthetic variations of the original content to expand its training scope. This helps trick the AI into learning from a broader range of scenarios without the need to capture more footage, maximizing the value of the datasets.
However, the quality of the initial video content within a dataset is a critical factor in AI upscaling effectiveness. High-quality source material can lead to a dramatic improvement—sometimes a two to fourfold increase—in the accuracy of the upscaled results compared to using datasets based on poor quality video.
The era from which the free video data is sourced can influence the AI's training. For instance, videos from different decades often reflect different production styles and technological limitations. This aspect can be beneficial, as it teaches AI to tailor its upscaling approach to meet modern viewing standards.
Free video datasets are particularly useful in enhancing algorithms that incorporate temporal coherence. This refers to the consistent flow of images across successive video frames. This is critical for achieving a seamless, more user-friendly viewing experience with upscaled video.
Currently, a number of researchers are exploring the benefits of combining multiple free video datasets. The idea is that integrating data from diverse sources can leverage their respective strengths. This approach is showing promise in the development of more robust AI models that are more versatile across diverse video genres and quality levels.
One of the key hurdles to using free downloadable video is the prevalence of noise within these datasets. Compression artifacts and other noise must be carefully filtered out via pre-processing steps to maximize the quality of training data for AI algorithms.
Interestingly, the ethical implications of using freely available video data are often overlooked. There is a growing need to discuss how to ensure datasets are truly open for use and avoid intellectual property violations. This remains a major point of debate within the AI research community.
Recent developments are showing signs that free video datasets could lead to real-time AI upscaling methods. As the algorithms become more computationally efficient, there's a good chance that video quality can be enhanced dynamically during streaming without requiring major hardware upgrades. This would fundamentally alter the way we watch video content.
The Impact of Free Downloadable Videos on AI Video Upscaling Techniques - Increased availability of high-quality training data enhances AI model performance
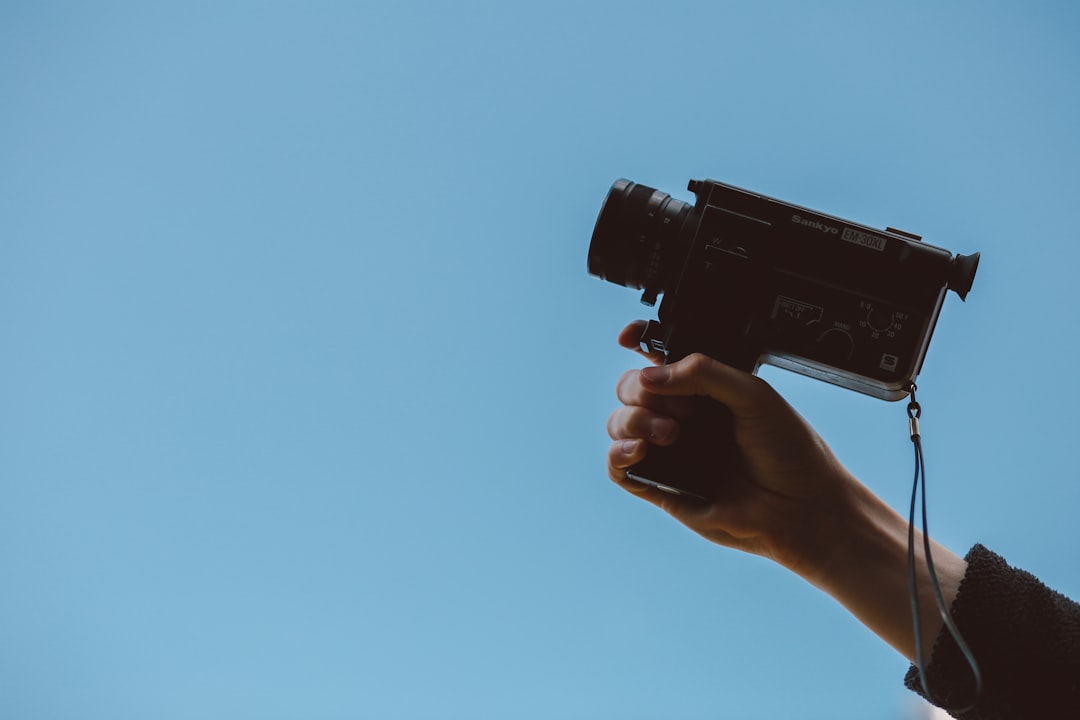
The abundance of high-quality training data is a driving force behind improvements in AI model performance, particularly within the field of video upscaling. The ability of AI to accurately upscale video relies heavily on the quality and diversity of the data used to train it. AI models trained on diverse video sources, encompassing a wide range of lighting conditions, motion patterns, and scene variations, demonstrate greater adaptability and generalization across different video types. However, the quality of the training data remains paramount. High-quality training data is essential for achieving high accuracy in upscaling, while lower-quality data can hinder performance and introduce artifacts. AI developers are also leveraging data augmentation techniques, where synthetic variations of existing video content are used to train models on a wider array of scenarios. This strategy is beneficial as it effectively expands the scope of training data without requiring additional real-world video capture. Ultimately, the careful selection and management of training data is critical for producing effective and robust AI video upscaling applications.
The increased availability of high-quality training data has been observed to positively impact the efficiency of training AI models. By reducing the computational resources required for training, it allows for more rapid experimentation with different model architectures, which is valuable for researchers and engineers trying to optimize their designs.
It's fascinating that AI models exposed to rich and varied training datasets often demonstrate a phenomenon called "transfer learning." This means the knowledge gleaned from one task can be readily applied to a different but related task, showcasing the power of diverse data in creating robust and adaptable AI systems.
However, it's crucial to avoid training datasets that lack sufficient variety. If the training data is too narrow in its scope, the resulting model may excel in that specific context but struggle when encountering different inputs. This can create highly specialized models that lack broader applicability.
Including datasets with a wide range of resolutions, even very low-quality ones, can be beneficial for upscaling algorithms. Exposure to different levels of compression artifacts can help the AI better understand how to reconstruct details in a way that minimizes errors.
Further, it's been observed that the inclusion of temporally diverse datasets—videos that capture a wide range of frame rates and movement types—can dramatically improve the visual consistency of upscaled video. Maintaining smooth transitions between frames is critical for maintaining high-quality video output.
Interestingly, even though it seems like a negative aspect, high levels of noise in training data can, in some cases, improve model robustness. By forcing the AI to sift through noisy information, it can lead to more resilient and adaptable feature extraction methods, potentially through a process called adversarial training.
Models trained on open-source video libraries have often demonstrated superior performance compared to those trained on proprietary datasets. This may indicate that the collaborative nature and inclusivity of open-source data can lead to unexpected breakthroughs in AI model performance.
When evaluating a dataset's effectiveness, the sheer number of attributes or features present within it, often referred to as dimensionality, can play a crucial role in determining how well the AI can generalize to new scenarios. Higher dimensional datasets generally tend to yield more insightful and adaptable models.
The well-known issue of overfitting, where an AI becomes overly specialized and can't adapt to variations beyond its training data, illustrates the need for balance. This suggests a mix of high and low-quality videos is useful to ensure a broader range of capabilities in the AI.
A noteworthy finding is that AI models can be effectively trained to "repair" videos. This ability to recover details lost due to severe compression or damage could be quite beneficial for preserving and making usable older or degraded media content.
The Impact of Free Downloadable Videos on AI Video Upscaling Techniques - Free video repositories enable broader testing of upscaling techniques
Openly available video collections are proving incredibly valuable for expanding the scope of how AI video upscaling techniques are tested. Researchers and developers can leverage these repositories' diverse video content to gauge the performance of various algorithms across a wide spectrum of scenarios and video types. This increased availability of diverse video data is particularly helpful for training AI models to better adapt to changing lighting, motion within scenes, and the visual characteristics of different types of video. While access to these repositories is a clear advantage for advancing the field, it's crucial to recognize that not all freely available videos are of high quality. Using poor-quality video can negatively affect the training process and potentially produce upscaled results with undesirable artifacts. Ultimately, the quality and variety of training data used to build these AI models will have a direct impact on how well they perform in the future.
The availability of numerous high-quality, free video repositories, encompassing amateur and professional recordings, provides AI researchers with diverse training sets that cover a range of video genres. This variety introduces both challenges and opportunities for machine learning models, forcing algorithms to adapt to different stylistic choices and video contexts. It's intriguing how these distinct aesthetic elements can influence the development of AI upscaling techniques.
The initial resolution of a video significantly impacts the AI's upscaling performance. Studies indicate that algorithms trained on high-resolution content tend to produce superior upscaled results compared to those trained on lower-quality sources. This disparity in performance emphasizes the importance of training data quality and its potential effect on visual improvements across various display technologies.
Surprisingly, the temporal aspects of video, specifically how frames evolve over time, can influence upscaling quality. AI trained with videos having consistent frame rates and predictable motion can better maintain visual integrity during upscaling. This is critical as it can help prevent issues like motion blur or artifacts that can often occur during dynamic scenes.
Preliminary research suggests that free video datasets can sometimes present a "curse of dimensionality"—where overly complex sets of features can confuse AI models. Striking the right balance in the number of training parameters is crucial to ensure that AI models can generalize well to different video types without compromising performance in specific applications.
A significant advantage of using public video datasets is the possibility of cross-validation across different AI models. This collaborative testing within open-source communities can accelerate the iteration process within video upscaling research, offering a clearer path toward developing more robust methodologies.
Interestingly, variations in video quality within a dataset can actually enhance the training process. When AI algorithms are exposed to both high-quality and low-quality footage, they develop a set of problem-solving strategies that allow them to more effectively reconstruct details and minimize artifacts in challenging video conditions.
The sheer volume of readily available video content has enabled researchers to conduct extensive experiments on different AI architectures without the usual costs associated with proprietary datasets. This increased research capability can lead to a faster pace of innovation in video upscaling technologies.
It's fascinating to observe how the prevalence of certain compression techniques across free video repositories can create a sort of pattern for AI models to learn. This knowledge of common compression artifacts allows algorithms to more readily predict and correct errors during upscaling, improving their ability to produce better results across a variety of video sources.
Machine learning techniques have shown promise not only for upscaling videos but also in enhancing the overall post-production process. As AI learns from these free video datasets, it increasingly automates tasks such as color correction and noise reduction, ultimately leading to smoother workflows for content creators.
While using free video datasets provides many benefits, the legal landscape surrounding these resources presents a set of opportunities and challenges. Ensuring compliance with intellectual property rights remains a crucial concern. Understanding copyright law is paramount for developers who wish to avoid legal complications while utilizing open-source content for AI training.
The Impact of Free Downloadable Videos on AI Video Upscaling Techniques - User-generated content expands the diversity of scenes for AI training
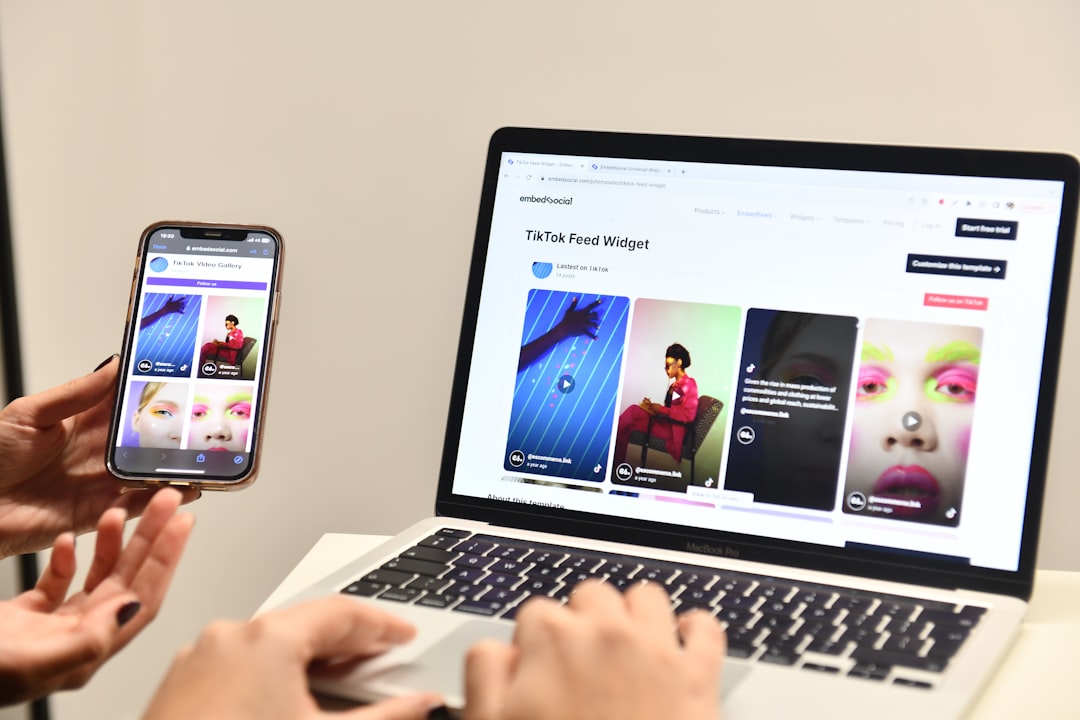
User-generated content (UGC) significantly broadens the range of visual scenes used to train AI systems. This diverse pool of videos, created by a wide variety of people, exposes AI models to a much wider array of shooting styles, locations, and subtle details. This exposure helps AI become more flexible and accurate in handling diverse video types. For example, AI algorithms trained on UGC can learn from a wider range of lighting situations, movement patterns, and scene compositions, aspects that might be poorly represented in more curated datasets. This broader training base not only helps refine video upscaling techniques but also raises questions about the originality and quality of AI-generated content. While the benefits of UGC are clear, it also introduces challenges, including the inconsistency of video quality and the ethical use of publicly available content.
User-generated content (UGC) offers a diverse range of visual scenes for AI training, encompassing everything from natural landscapes to urban environments. This variety is key for AI algorithms to develop a deeper understanding of context and how scenes typically unfold. It's quite interesting that UGC exposes AI to a wider array of cultural and social settings, fostering a more global perspective on video styles and preferences, which could be reflected in the quality of the upscaled videos.
The involvement of both amateur and professional content creators in UGC introduces variety in video quality, which, in turn, helps AI algorithms create more versatile methods for restoring quality. Surprisingly, UGC often captures spontaneous moments and unconventional camera angles, a valuable trait for preparing AI for real-world scenarios where videos might not adhere to conventional formats.
Furthermore, the inherent temporal continuity within UGC—how scenes change over time—provides a training ground for AI models to grasp motion dynamics, a crucial aspect for preserving visual quality during upscaling. This can minimize common motion artifacts that negatively impact the viewing experience.
UGC often contains localized content and specialized scene types, which might not be common in professionally curated datasets. This allows AI systems to adapt to these specialized scenarios, potentially increasing their overall performance. User-generated content often reflects current trends and informal filming techniques, so AI upscaling methods need to be capable of adapting to evolving video production practices and technologies.
UGC encompasses a broad spectrum of resolutions, from high-definition to low-quality recordings. This diversity equips AI algorithms to better reconstruct details across various levels of video quality, resulting in more reliable and versatile upscaling techniques.
Beyond the technical aspects, UGC's collaborative nature fosters a sense of community amongst content creators, where insights and techniques are shared, which can influence AI training and the development of new upscaling methods.
It's important to remember that the inconsistent quality in UGC can present hurdles. Poorly captured videos within a training dataset can undermine the effectiveness of AI algorithms. AI developers need to curate UGC strategically to ensure they extract the maximum potential for high-quality video enhancements.
The Impact of Free Downloadable Videos on AI Video Upscaling Techniques - Open-source video collections accelerate research in upscaling methods
Open-source video collections are proving to be a powerful catalyst for research into video upscaling methods, particularly those driven by artificial intelligence. These collections provide a vast and diverse range of training data, allowing researchers to experiment with and refine their AI algorithms across a wide variety of video types and conditions. This helps AI models become more versatile and effective at improving video resolution. However, the quality of these datasets can be inconsistent, and poor-quality videos can negatively impact the training process, potentially leading to upscaled videos with undesirable visual flaws. Successfully leveraging these open-source collections requires a delicate balance between the benefits of wide-ranging diversity and the need for quality control, as a carefully curated dataset is crucial for maximizing the accuracy and effectiveness of AI upscaling techniques. In essence, the growing availability of open-source video data both presents a wealth of opportunities and introduces complexities for the future development and refinement of AI-based video enhancement tools.
Open-source video collections aren't just beneficial for enhancing the accuracy of AI upscaling models, they're also a catalyst for innovation in developing new algorithms. By offering diverse video scenarios for testing, researchers can push the boundaries of what's possible.
Some video collections have a range of compression levels, which helps AI systems learn how to manage and fix compression artifacts. This leads to better overall upscaling quality as the AI becomes better at recognizing and dealing with different types of artifacts.
Interestingly, AI models trained on a mix of user-generated and professionally-created videos seem to become more adaptable. These hybrid training sets expose the AI to both unique user-created styles and the consistent quality of professionally made videos, improving performance with new and unexpected input.
User-generated content (UGC) often contains real-world complexities like shaky camera work and challenging lighting situations. These challenges encourage AI to develop more robust and versatile approaches to handle unpredictable environments.
The consistent flow of images across video frames in UGC helps AI models better understand motion dynamics. This understanding improves their ability to preserve detail even in fast-paced scenes without introducing distracting artifacts.
Some AI upscaling models trained using community-contributed datasets have surprisingly developed the ability to independently reconstruct details in low-quality or heavily compressed footage. This is an accomplishment that would be hard to achieve using only high-resolution training data.
A potential hurdle, although not often discussed, is the reliance on the specific platform or social media site where UGC is stored. If these platforms have restrictions on the kinds of videos that are shared, this could limit the diversity required for effective AI training.
Training with video datasets that include a wide variety of settings – rural, urban, and unique cultural environments – allows upscaling algorithms to be adapted for broader audiences. It’s fascinating how these diverse sources expose the nuances in content that can impact how we perceive video quality.
The collaborative nature of open-source communities in creating and curating these video datasets speeds up the feedback process. This dynamic helps developers refine their algorithms more quickly based on real-world challenges that users encounter.
While the benefits of using massive video collections are significant, it’s important to remember the need for pre-processing steps to remove noise and irrelevant content. Neglecting this crucial step can lead to a noticeable drop in the performance of AI upscaling models.
The Impact of Free Downloadable Videos on AI Video Upscaling Techniques - Ethical considerations arise from using freely shared videos for AI development
The increasing use of freely shared videos for AI development introduces a range of ethical concerns that demand attention. Using these readily available datasets can potentially compromise individual privacy, particularly if personal information is unknowingly incorporated into the training data. Moreover, the diverse and often inconsistent quality of freely available videos can introduce biases and authenticity issues into the AI's output, potentially leading to skewed or misleading results. The use of these datasets also raises legal concerns, as there's a risk of violating intellectual property rights if not handled carefully. These issues underscore the crucial need for establishing clear ethical guidelines that govern the acquisition, use, and output of AI systems trained on openly shared video content. This is essential to ensure that the development and deployment of AI in this domain is done responsibly and with due consideration for potential harms.
Utilizing freely shared videos for AI development introduces a range of ethical considerations we need to carefully examine. The legal landscape surrounding these videos is intricate, with potential copyright infringement issues that could result in legal battles if not handled correctly. Developers must diligently ensure their actions align with copyright laws to steer clear of any legal trouble.
Understanding the origins of a dataset is crucial. Videos that appear freely available might have hidden restrictions we might not initially recognize. This can include copyright claims or licensing agreements that limit how the data can be employed in AI model training, creating unexpected roadblocks.
The ethical use of user-generated content (UGC) in AI model training raises questions about consent and ownership. Many individuals who contribute to these open platforms might not be aware their videos are being utilized to build commercial products or services, which can be concerning.
Despite the valuable diversity offered by UGC, it often lacks the stringent quality controls seen in curated datasets. This can lead to biases within the AI systems. The AI might misrepresent certain groups or cultures if it's trained primarily on certain kinds of UGC, revealing the importance of dataset curation.
The uneven quality of readily available video data can introduce inconsistencies during AI training. This can result in AI models that become overly reliant on the characteristics of lower-quality data. Consequently, they might struggle to deliver optimal results when faced with higher-quality video inputs, underlining the significance of carefully crafting datasets.
Ethical considerations caution against oversimplifying video content to meet the requirements of AI models. This homogenization can inadvertently erase the unique qualities of cultural expressions found in diverse videos, potentially impacting the authenticity of AI-generated outputs.
The presence of noise and visual artifacts in many freely accessible video datasets can considerably hinder AI performance. To mitigate these challenges, robust pre-processing techniques are essential before AI training commences.
An often-overlooked aspect is the impact of obsolete or culturally irrelevant videos in freely available repositories. These older videos can unintentionally influence AI preferences toward outdated styles and trends, potentially distorting the evolution of contemporary video production aesthetics.
The cooperative nature of open-source repositories can sometimes lead to an unchecked growth of low-quality videos. This highlights the need for community-driven efforts to strike a balance between fostering diverse content and upholding quality standards.
Ethically employing free video datasets necessitates continuous discussions about the consequences of using these shared resources. This compels us to rethink how community-generated content can positively influence AI development while acknowledging the rights of the original content creators.
Upscale any video of any resolution to 4K with AI. (Get started for free)
More Posts from ai-videoupscale.com: