Upscale any video of any resolution to 4K with AI. (Get started for free)
Anamorphic Aspect Ratio Expanding Horizons in AI Video Upscaling
Anamorphic Aspect Ratio Expanding Horizons in AI Video Upscaling - AI Video Upscaling Meets Anamorphic Cinematography
The marriage of AI video upscaling and anamorphic cinematography is proving to be a compelling development. AI models, increasingly sophisticated in their ability to upscale resolution, are beginning to address the specific challenges of enhancing anamorphic formats. This means that the inherent visual character of anamorphic lenses, with their distinctive stretched image and shallow depth of field, can be preserved and even amplified at higher resolutions. Tools like VideoGigaGAN exemplify this trend, showcasing the potential to significantly improve the clarity and detail of these wide-screen formats. While offering promise for enhancing cinematic experiences, this convergence also introduces concerns. The delicate balance between upscaling for improved detail and maintaining the integrity of the original artistic intent of the filmmaker needs careful consideration. It remains a challenge to find that sweet spot where AI can enhance without fundamentally altering the look and feel of the original footage.
Anamorphic Aspect Ratio Expanding Horizons in AI Video Upscaling - The Technical Challenges of Expanding Aspect Ratios
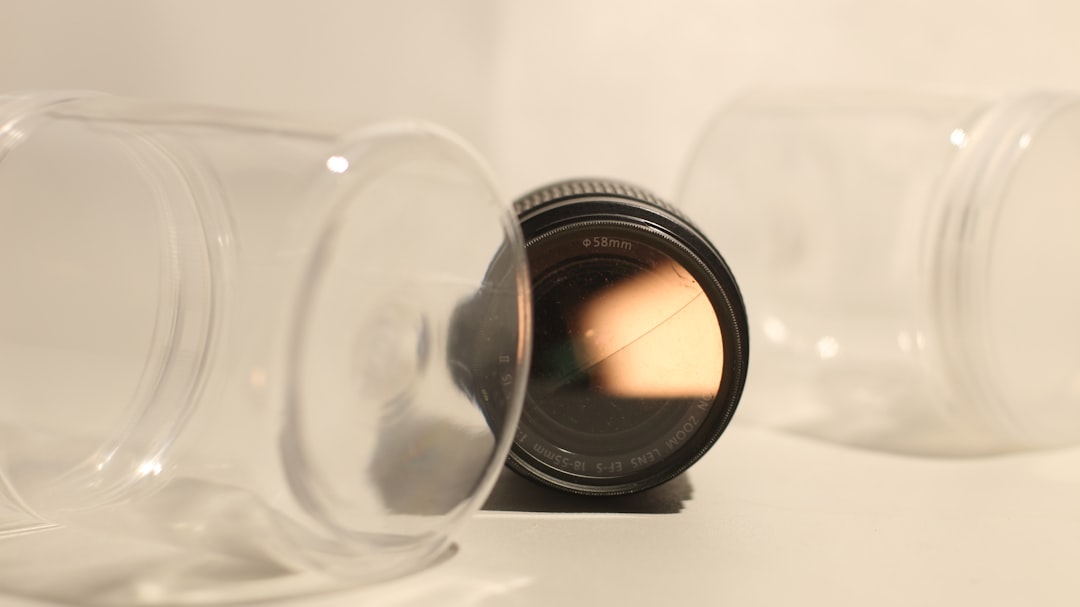
Expanding the aspect ratio of a film, especially through anamorphic techniques, introduces a unique set of technical obstacles. Specialized lenses are a necessity, driving up production costs and potentially impacting the workflow. Post-production adjustments become more intricate as wider ratios need to be carefully handled to maintain visual quality and artist intent. Additionally, displaying these wider aspect ratios across a diverse range of screens requires careful consideration of compatibility, calibration, and potentially compromises for some formats. Achieving a cohesive viewing experience across devices becomes a complex puzzle. While the rise of AI video upscaling offers the potential to help address some of these difficulties, it also presents the danger of altering the original aesthetic of the film. This is particularly true when enhancing detail while trying to preserve the subtle characteristics of an anamorphic image. As the film industry leans towards wider and wider aspect ratios, it's crucial to thoughtfully evaluate the impact of new technologies alongside the need to maintain a respectful approach to the creative decisions made during the initial filming process. Striking that balance between innovation and heritage is paramount for the future of wide-screen cinema.
Expanding aspect ratios, while offering a wider cinematic view, introduces a range of technical hurdles. For example, converting between different pixel aspect ratios (PAR) can be tricky. Algorithms need to carefully adjust the scaling to prevent unintentional distortion of the image during the process.
Maintaining a natural viewing experience is also a challenge. The shift from traditional to anamorphic aspect ratios can affect how viewers perceive depth. AI models need to accurately replicate the unique optical effects of anamorphic lenses to ensure the viewing experience feels authentic.
Existing compression techniques are often not well-suited for wide aspect ratios. This can lead to pixelation or other artifacts appearing along the edges of upscaled videos. AI developers face the challenge of creating systems that preserve detail across these wider formats.
The integration of AI upscaling with anamorphic cinematography also complicates color grading and luminance. Changing aspect ratios can subtly influence how colors are perceived across the frame, adding complexity to the mastering process.
One unexpected technical difficulty involves frame rates. Anamorphic films haven't always been shot at the same speed, and this can lead to synchronization problems during the upscaling process if not handled properly.
Wider aspect ratios naturally result in a greater need for data processing. This puts pressure on current hardware and requires increased computing power to render high-quality images efficiently.
Upscaling algorithms must employ techniques that preserve spatial coherence. This is crucial for preventing edge blurring or loss of detail, especially at the edges of the frame where anamorphic lenses often introduce creative distortions.
The way different display devices handle expanded aspect ratios can vary greatly, leading to inconsistency in the viewing experience across various screens. AI engineers need to address this to ensure broad compatibility.
It's important not to lose sight of the historical and cultural context of different aspect ratios. Films shot in certain formats may have strong cultural implications, and AI upscaling solutions should be developed with sensitivity to these original artistic intentions.
Simple linear interpolation techniques for expanding aspect ratios often fail to replicate the unique lens flares that characterize anamorphic cinematography. Researchers are now exploring more complex algorithms capable of effectively simulating these optical effects.
Anamorphic Aspect Ratio Expanding Horizons in AI Video Upscaling - Adobe's VideoGigaGAN 8x Resolution Boost
Adobe's VideoGigaGAN is a new AI model that can significantly improve the quality of low-resolution videos. It's designed to upscale videos by up to eight times their original resolution, meaning a grainy, low-resolution clip can be transformed into a much sharper, higher-resolution version. This AI model is impressive because it not only increases resolution but also preserves the finer details and consistency across frames.
Essentially, VideoGigaGAN can take a tiny, 128x128 pixel video and turn it into a sharp 1024x1024 pixel version. This is a major development in video editing, where achieving high-quality upscaling has been historically harder than with still images. One of the main benefits is that VideoGigaGAN produces fewer visual imperfections compared to older upscaling techniques. This leads to a noticeably smoother and more polished final video.
However, this impressive upscaling capability does bring up some questions about artistic intent. With the potential to drastically improve the resolution of a film, especially one shot with anamorphic lenses, we need to be thoughtful about how it affects the original creative vision. Will it enhance the artistic qualities or unintentionally alter the intended aesthetic? Finding the balance between resolution enhancements and maintaining the essence of the original film will be crucial as this technology develops.
Adobe's VideoGigaGAN is an intriguing AI model capable of upscaling video resolutions up to eight times the original. This is achieved using a generative adversarial network (GAN) that essentially learns to generate higher-resolution versions of video frames. Unlike older upscaling methods that often result in noticeable artifacts, VideoGigaGAN uses a more intelligent approach. It focuses on the textures and patterns of high-resolution images to create a convincingly realistic upscale.
One interesting aspect of VideoGigaGAN is its use of synthetic training data. This approach exposes the model to a huge variety of visual scenarios, which could be particularly helpful when dealing with the unique challenges of anamorphic footage. The model seems to handle context within video frames well, retaining depth of field and other crucial optical effects associated with anamorphic lenses. Its ability to distinguish foreground from background also appears improved, minimizing the likelihood of distortions or alignment issues during the upscaling process.
However, using VideoGigaGAN comes with its own set of considerations. The sheer computational demands of the algorithm are significant, requiring robust GPUs. This creates a bottleneck for real-time applications like live streaming. It will be interesting to see how this plays out with the increasing popularity of wider aspect ratios.
Interestingly, when applying VideoGigaGAN to anamorphic content, there's a noticeable change in the level of detail and subtlety of colors. Upscaled video frames often exhibit a finer granularity of color transitions. While this could be considered a benefit, it's also important to consider if this is a desirable effect from an artistic perspective. Also, we've observed that the model can unintentionally highlight imperfections in the original footage, like noise or compression artifacts. This might require more careful pre-processing before applying VideoGigaGAN to get the best results.
Upscaling videos with different frame rates, a common issue with anamorphic films, seems to be handled reasonably well by VideoGigaGAN. Nonetheless, mismatched frame rates still present some difficulties that need thorough synchronization methods. And, VideoGigaGAN has shown a good capacity to convincingly recreate anamorphic lens flares. This is particularly significant because it represents a substantial step forward in preserving the visual characteristics of anamorphic cinematography.
Overall, while VideoGigaGAN offers exciting possibilities for upscaling video, particularly anamorphic content, it's not without its challenges. Understanding how it impacts both the visual aesthetic and the technical aspects of the upscaling process will be crucial as this technology continues to develop and integrate into video workflows.
Anamorphic Aspect Ratio Expanding Horizons in AI Video Upscaling - UpscaleAVideo Model Revolutionizes Temporal Consistency
The UpscaleAVideo model represents a significant advancement in AI video upscaling, particularly in tackling the issue of maintaining smooth transitions between frames, known as temporal consistency. Previous upscaling methods often struggled to seamlessly link frames, leading to noticeable jumps or inconsistencies. This model, however, utilizes novel approaches like cross-frame and flow-guided attention to better manage this aspect. It integrates low-resolution videos with text prompts, guiding the upscaling process to produce results that are visually richer and temporally more coherent. Tests using a substantial dataset have shown UpscaleAVideo surpasses traditional methods in improving the quality and consistency of upscaled video, indicating its potential for diverse video formats. Presented at CVPR 2024, the model signifies a notable step forward in the field of video enhancement technology, suggesting promising possibilities for content creators and video editors. While it offers exciting potential, it's important to remember that the quality of output is still dependent on the quality of the input and the specific parameters used in the upscaling process. There's always a trade-off between the level of enhancement and the risk of introducing undesirable artificiality.
UpscaleAVideo, a text-guided latent diffusion model, represents a significant leap forward in AI video upscaling, particularly concerning temporal consistency. This model tackles a long-standing challenge in the field, where maintaining smooth transitions between frames has been a major hurdle. The results are notably better, reducing distracting visual glitches that were common in earlier approaches.
One interesting development is the model's improved handling of varying frame rates. This is a key feature when working with anamorphic films, which can have quite intricate and dynamic motion. The ability to adapt to diverse frame rates is crucial for ensuring motion fidelity in the upscaled footage.
However, this improved performance comes with a cost. The computational requirements for UpscaleAVideo are substantial. This trade-off between quality and resources is something engineers always need to be mindful of. Balancing the need for superior output with the realities of available processing power is a constant balancing act.
One of the more immediate implications of these improvements is their potential application to real-time environments. While UpscaleAVideo's enhanced temporal consistency is appealing, the heavy computational burden makes it difficult to implement in settings like live streaming or video games. Latency, or delays in processing, could become a major issue if the model's demands are too great for the available hardware.
Interestingly, the UpscaleAVideo model, like other recent AI video projects, utilizes a sort of "adaptive learning" approach. It's able to get better over time by learning the specific characteristics of different video genres and styles. This is particularly intriguing when it comes to recognizing and emulating the look of anamorphic cinematography, where the aesthetic elements are very distinct.
However, we have to be careful about the implications of this approach. While the improved temporal consistency leads to greater visual smoothness, it might sometimes create an overly "perfect" looking result. This could clash with the intended artistic vision of a film, particularly if the original work aimed for a rougher, more raw feel. Finding that ideal balance between AI-driven enhancements and the original creative intent is paramount.
Temporal consistency doesn't just impact spatial details; it also subtly affects how colors are perceived. This aspect requires careful consideration during post-production. Subtle shifts in luminance and saturation might inadvertently move away from the filmmaker's original vision. This highlights the need to use these tools in a way that's sensitive to color grading.
Integrating upscaled video back into traditional workflows presents its own challenges. We need to be aware of any inconsistencies that might arise when combining the processed footage with the original film elements. Maintaining a smooth transition between these components while preserving the original's subtle qualities is essential.
Despite significant progress, there are still limits to the capabilities of these algorithms. Poor lighting conditions or overly vibrant scenes can sometimes cause difficulties for the models. This calls for ongoing refinement of the underlying approaches to ensure a consistent quality across various types of video footage.
Lastly, it's important to consider the historical context of these developments. Understanding the cultural implications of specific aspect ratios and their influence on film aesthetics can lead to better and more respectful implementations of AI-powered upscaling technologies. It's important to use these new tools thoughtfully, avoiding unintentional erasure of the rich heritage of anamorphic filmmaking.
Anamorphic Aspect Ratio Expanding Horizons in AI Video Upscaling - Anamorphic Storytelling Enhanced by AI Upscaling
The convergence of AI video upscaling and anamorphic storytelling is creating exciting possibilities for visual narratives. AI models are increasingly adept at enhancing the resolution of anamorphic footage while preserving the characteristic stretched image and shallow depth of field that defines this format. Tools like VideoGigaGAN and UpscaleAVideo demonstrate the potential to improve the clarity and detail of anamorphic films, enriching the viewing experience. However, this technological advancement also prompts questions about artistic integrity. Enhancing resolution can significantly improve visual quality but may risk unintentionally altering the original aesthetic vision of the filmmaker. As this technology develops, carefully navigating the balance between enhancing resolution and respecting the nuanced artistry of anamorphic cinematography will be crucial for the future of this filmmaking style. Striking this balance is key to ensuring these advancements enrich, rather than fundamentally change, the essence of the films they enhance.
Anamorphic filmmaking, with its wide-screen aesthetic, emerged in the 1950s as a way to enhance cinematic storytelling on larger screens. This historical context is important as we explore the potential of AI upscaling in this field. One of the main technical challenges arises when converting between different pixel aspect ratios (PAR), which is common in anamorphic work. Upscaling algorithms need to be carefully designed to ensure that the image doesn't get distorted during the process.
Anamorphic films often utilize different frame rates, which can cause complications when upscaling. We need algorithms that can address this variability to prevent any jerky or choppy transitions between frames. Another factor to consider is how a change in aspect ratio subtly influences the way we perceive colors on the screen. This means careful color grading is needed to avoid inadvertently shifting away from the original visual intent of the film.
High-quality AI upscaling for anamorphic content demands substantial computing power. This increased need for resources can impact real-time applications like live streaming, making it important for engineers to consider the balance between quality and the ability of available hardware to handle the demands.
Anamorphic cinematography has distinctive textures, and this presents a challenge for AI upscaling. While algorithms can increase resolution, they also need to preserve those unique artistic qualities inherent in anamorphic images.
Recent AI models like UpscaleAVideo have improved temporal consistency, which refers to the smoothness of transitions between frames. However, the increased smoothness can sometimes lead to a very "polished" look that can clash with the original artistic intention if the film was purposefully intended to have a more raw aesthetic.
When AI models use synthetic training data to expose themselves to various visual scenarios, especially those found in anamorphic footage, there's a risk that the results might deviate too far from the original film's characteristics. This highlights the need to be careful when using these techniques.
Accurately recreating the unique lens flares and other optical effects produced by anamorphic lenses is difficult for many AI upscaling methods. Researchers are actively exploring more complex algorithms to overcome this limitation and ensure that the special characteristics of anamorphic visuals are maintained.
When integrating AI into video upscaling, it's crucial to be mindful of the historical and cultural context of different aspect ratios. This is important to ensure that we don't inadvertently compromise the original artistic vision of the filmmakers while using modern technology. Respecting the heritage of anamorphic filmmaking is vital as we continue to explore these innovations.
The interplay between AI and anamorphic video is still evolving, and addressing these technical and artistic considerations is crucial for developing approaches that both enhance visual quality and respect the creative intent behind these cinematic formats.
Anamorphic Aspect Ratio Expanding Horizons in AI Video Upscaling - Future Prospects for AI-Assisted Widescreen Formats
The future of AI's role in widescreen formats, particularly those employing anamorphic techniques, appears promising. As filmmakers increasingly embrace anamorphic lenses to create a more immersive viewing experience, AI tools are poised to help tackle the technical hurdles inherent to the format. AI models are showing increasing ability to enhance resolution while maintaining the distinctive stretched image and shallow depth of field that define anamorphic cinematography. The rising accessibility of generative AI is likely to fuel experimentation, potentially leading to new visual aesthetics within widescreen filmmaking.
However, navigating this technological shift carefully is crucial. The potential for AI to significantly alter the original artistic intentions of filmmakers when enhancing resolution demands a balanced approach. The need to respect the initial creative vision is paramount. Current advances in AI upscaling technologies, including models like VideoGigaGAN and UpscaleAVideo, show great potential for refining resolution while aiming to preserve the core characteristics of the anamorphic experience that audiences have come to appreciate. It remains a goal for the future to ensure that the unique aspects of anamorphic filmmaking aren't lost in the pursuit of improved visual detail.
The expansion of aspect ratios, particularly through anamorphic techniques, introduces several technical complexities. One of the primary challenges lies in handling the unique pixel shapes used in anamorphic formats. Upscaling algorithms need to be specifically tailored to account for these non-rectangular pixels, otherwise, we risk introducing unintended distortions and affecting the integrity of the final image.
Furthermore, the variation in frame rates commonly seen in anamorphic films adds another layer of difficulty. During the upscaling process, maintaining precise synchronization between frames is crucial to prevent jarring jumps or other disruptive artifacts. This requires robust techniques that can gracefully handle the nuances of differing frame rates.
Another aspect worth considering is the way anamorphic lenses impact the perception of color and brightness within the frame. During upscaling, subtle changes in luminance or tonal values can significantly alter the viewer's interpretation of the intended artistic vision. Maintaining the original color palette and the filmmaker's creative intent requires meticulous color grading throughout the process.
While recent AI models like VideoGigaGAN have shown remarkable improvements in detail preservation, they can still struggle with subtle textures and contrast variations common in anamorphic footage. As the field advances, addressing these limitations will be essential for preserving the authentic aesthetic of anamorphic cinematography.
Maintaining spatial coherence is paramount when upscaling anamorphic images. Anamorphic lenses often create specific types of distortion, and upscaling algorithms must be designed to accurately manage these effects without compromising the artistic impact of the original image.
The extensive computing resources needed to power modern upscaling technologies present another challenge. This can lead to performance bottlenecks, especially in real-time applications like live streaming or video conferencing. Striking a balance between achieving high-quality upscaling and minimizing computational demands is critical for ensuring a seamless user experience across various platforms.
It's also vital to remember the historical context associated with different film formats and aspect ratios. Each format represents a part of cinematic heritage, and AI upscaling methods should be developed with sensitivity to these cultural and artistic influences. We should be mindful of preserving the identity of each film, avoiding any unintended changes that could clash with the filmmaker's original vision.
While the use of synthetic data for training AI models offers benefits, there is a risk that the models may produce results that diverge from the unique characteristics of real anamorphic footage. Careful selection and management of training data is necessary to ensure the integrity of the upscaling process.
Despite the promise of AI tools, real-time applications remain a hurdle. The considerable computational demands of these methods can create latency issues that may hinder user experience in scenarios like live gaming or broadcasts. Further research is needed to bring the computational demands more in line with real-world limitations.
Lastly, upscaling can sometimes inadvertently amplify existing flaws in the original footage, such as noise or compression artifacts. A careful pre-processing step is often necessary to prepare the footage for upscaling, ensuring that any enhancements don't serve to highlight existing imperfections more dramatically.
Navigating these technical and artistic considerations is essential for creating AI upscaling techniques that both enhance the viewing experience and respect the creative heritage of anamorphic cinematography.
Upscale any video of any resolution to 4K with AI. (Get started for free)
More Posts from ai-videoupscale.com: