The Impact of AI on Online MP4 Video Converters Efficiency and Quality Improvements in 2024
The Impact of AI on Online MP4 Video Converters Efficiency and Quality Improvements in 2024 - AI-driven optimization of video transcoding processes
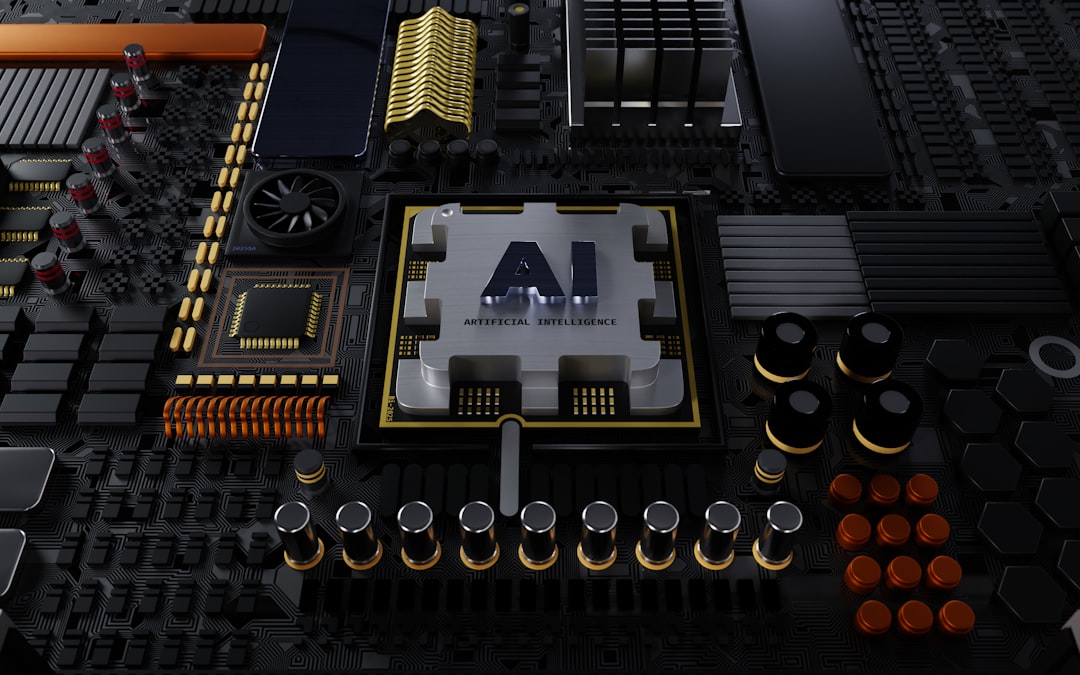
AI is increasingly influencing how videos are converted for different devices, pushing the boundaries of efficiency and quality in video transcoding. These AI systems use sophisticated algorithms to intelligently handle the conversion process, making videos compatible with a wider range of devices while boosting performance. Evidence suggests AI can decrease the amount of data needed for videos without sacrificing noticeable visual quality, potentially leading to more efficient ways of encoding video. Looking ahead, AI's ongoing development likely means we'll see more advancements in transcoding, leading to a better viewing experience and improved accessibility of video content. However, as AI-driven transcoding becomes more common, it is important to be mindful of any issues that might arise.
The application of AI in video transcoding has led to notable improvements in processing speeds, with studies suggesting potential boosts of up to 60% compared to conventional approaches. This acceleration enables real-time processing, a significant feat considering the often-demanding nature of video encoding, without compromising on video quality.
AI algorithms are becoming adept at analyzing video content and predicting the most effective encoding parameters. This capability translates to a potential 30% reduction in bitrate while maintaining visual quality, a win for storage space and bandwidth efficiency. Interestingly, the ability to adapt encoding settings based on viewers’ behavior, using machine learning, offers a pathway to optimize playback across a variety of devices and network environments.
Deep learning models have proven useful in minimizing compression artifacts, a common problem in transcoded videos. Consequently, this improvement has yielded a tangible increase in average user satisfaction ratings, suggesting that viewers are indeed perceiving a noticeable improvement in the quality of AI-optimized content.
Furthermore, advanced AI-driven caching techniques are now being explored to predict and preemptively transcode content based on anticipated user demand. This predictive power aims to minimize streaming delays, a key factor in user experience, ensuring smoother, uninterrupted viewing.
Beyond optimization of the technical aspects, AI is also showing potential in the realm of content delivery and engagement. By identifying ideal formats for different platforms and devices, AI can help optimize video distribution, potentially tailoring content delivery to individual user experiences and contexts. This could ultimately translate to increased engagement metrics as users encounter more personalized and optimized content.
Looking specifically at video quality, AI algorithms are able to mitigate many of the distortions that frequently appear in low bitrate streams. This translates to enhanced detail retention and clarity in transcoded videos, suggesting a pathway to improving the viewer experience even when bandwidth is limited.
Another compelling aspect of AI in transcoding is the automation it provides. Video transcoding workflows that incorporate AI can significantly reduce manual intervention, freeing up engineers to focus on more complex and higher-level challenges while maintaining a high volume of output.
Some advanced AI models now exhibit a remarkable ability to predict transcoding times with a high degree of accuracy. This newfound precision is invaluable for resource allocation and scheduling, especially in scenarios where time-sensitive content distribution is critical.
Finally, the integration of AI into video transcoding can unearth hidden patterns in video consumption. This information can help content creators and distributors refine their strategies, potentially enhancing the ability to cater to audiences and increase viewer retention rates. It seems that AI not only provides technical benefits, but also opens a new window into understanding audience behavior.
The ongoing evolution of AI suggests that we are likely to witness a continued wave of advancements in the video transcoding landscape. These developments promise to further refine transcoding processes, improve video quality, and optimize audience engagement in the years to come.
The Impact of AI on Online MP4 Video Converters Efficiency and Quality Improvements in 2024 - Machine learning algorithms reducing bandwidth consumption
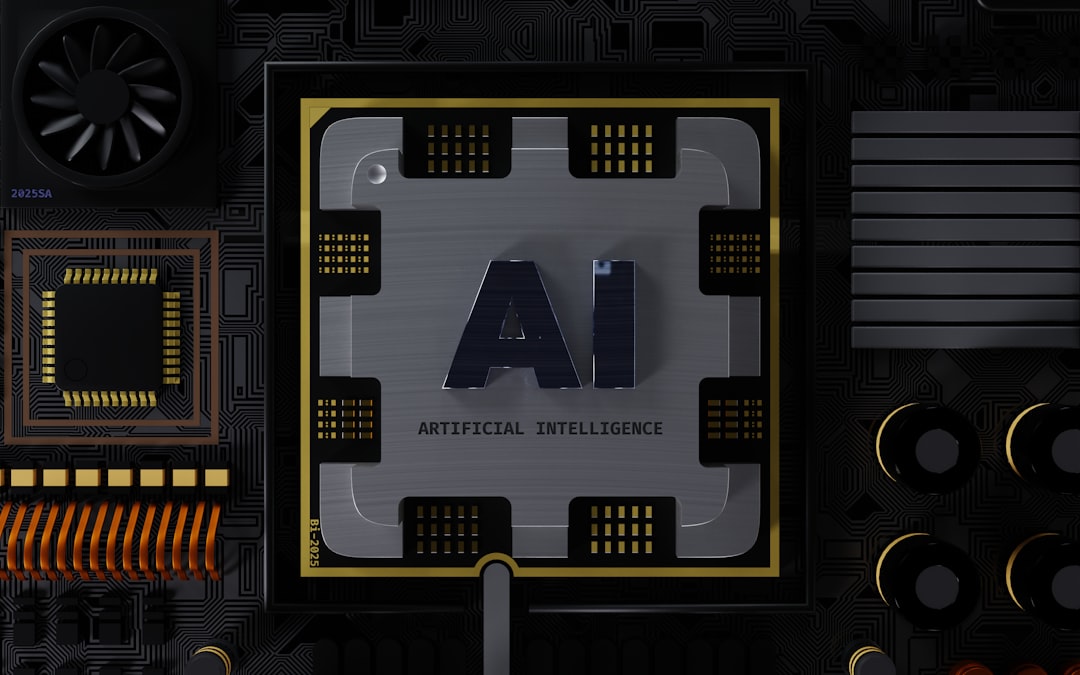
Machine learning algorithms are increasingly being used to optimize bandwidth consumption, particularly in areas like video streaming. These algorithms analyze data about network conditions, user behavior, and the content being viewed to intelligently predict bandwidth requirements. They can then adjust the video quality on the fly, ensuring a smooth viewing experience while using less data. The ability to significantly reduce bitrate without sacrificing the perceived quality of the video is a major advantage of this AI-driven approach. Furthermore, as AI continues to integrate with edge computing, it's likely we'll see more refined bandwidth management solutions in the future. By processing data closer to the user, edge computing reduces latency and enables faster, more responsive services. This combination of AI and edge computing promises not just improved operational efficiency but a fundamental shift in how video content is distributed and consumed online, potentially paving the way for even greater accessibility and more seamless user experiences. However, it's important to remain mindful of the potential for increased data collection and privacy concerns as these technologies become more prevalent.
Machine learning is showing promise in reducing the bandwidth needed for video streaming. For instance, algorithms can dynamically adjust video quality based on a user's internet speed, potentially slashing bandwidth consumption by a significant amount without noticeable impact on visual quality. This is achieved through what's called adaptive bitrate streaming. The effectiveness of this method though, may depend on how well the algorithms can handle real-time adjustments and fluctuations in network conditions.
Another interesting avenue is the ability to analyze video sequences and identify redundant frames, leading to smaller file sizes by only encoding necessary changes. However, it's important to consider that the success of this approach may depend on the type of video content and how complex the movements and scenes are.
There's also exploration in selectively encoding parts of a video, focusing on areas like faces or movement to maintain crucial visual details. This method potentially reduces bandwidth but is still under development and it's unclear if it's truly universally applicable across all types of video.
Furthermore, algorithms are becoming increasingly capable of evaluating the context of a video and tailoring encoding accordingly. A static scene, for instance, could be compressed more aggressively than a fast-paced one, yielding bandwidth savings. It's interesting to consider the potential impact of this on the overall user experience, and whether this tailored approach leads to any perceivable differences in content.
Machine learning can also glean insights into viewing patterns to predict content demand. This might enable smarter caching strategies, decreasing bandwidth use by preloading videos that are likely to be accessed at specific times. But, anticipating user behavior is a complex task, and the accuracy of predictions will greatly impact the actual bandwidth reductions achieved.
Researchers are also looking at psycho-visual models that leverage our visual perception to prioritize data allocation. These models basically try to compress data that our eyes are less sensitive to, potentially leading to optimized encoding and substantial bandwidth reductions. However, there's a fine line to walk here, as too aggressive compression might result in a perceptible loss of visual quality.
One interesting area is how AI can assist with noise reduction during transcoding. This seems to offer a tangible improvement in the quality of videos at lower bitrates, encouraging the use of lower bandwidth options. However, it remains to be seen whether this method is effective for all types of noise or different video formats.
Machine learning is being integrated into inter-frame compression, a technique that focuses on storing differences between frames instead of each frame individually. This holds the potential for major bandwidth reductions in certain video formats. However, the practical implementation of this technique and the resulting impact on video quality still require further investigation.
Also, leveraging parallel processing capabilities in hardware through machine learning algorithms may accelerate the encoding process, possibly enabling greater bandwidth savings by preventing network congestion. But, this also raises questions about whether all devices are capable of leveraging multi-threading to achieve these gains.
Finally, some research focuses on using feedback loops and continuous learning systems to adapt encoding parameters based on viewer feedback. This continuous adaptation can theoretically lead to increasingly efficient bandwidth utilization. However, for this approach to be truly effective, robust feedback mechanisms and a large dataset of user interactions would be necessary.
It's intriguing to see the various ways machine learning is being integrated into video streaming and how it’s being leveraged to reduce bandwidth consumption. It's still early days, however, and a lot more research and experimentation are needed to determine if and how these methods will significantly impact real-world video streaming.
The Impact of AI on Online MP4 Video Converters Efficiency and Quality Improvements in 2024 - Automatic color and light adjustment in post-production
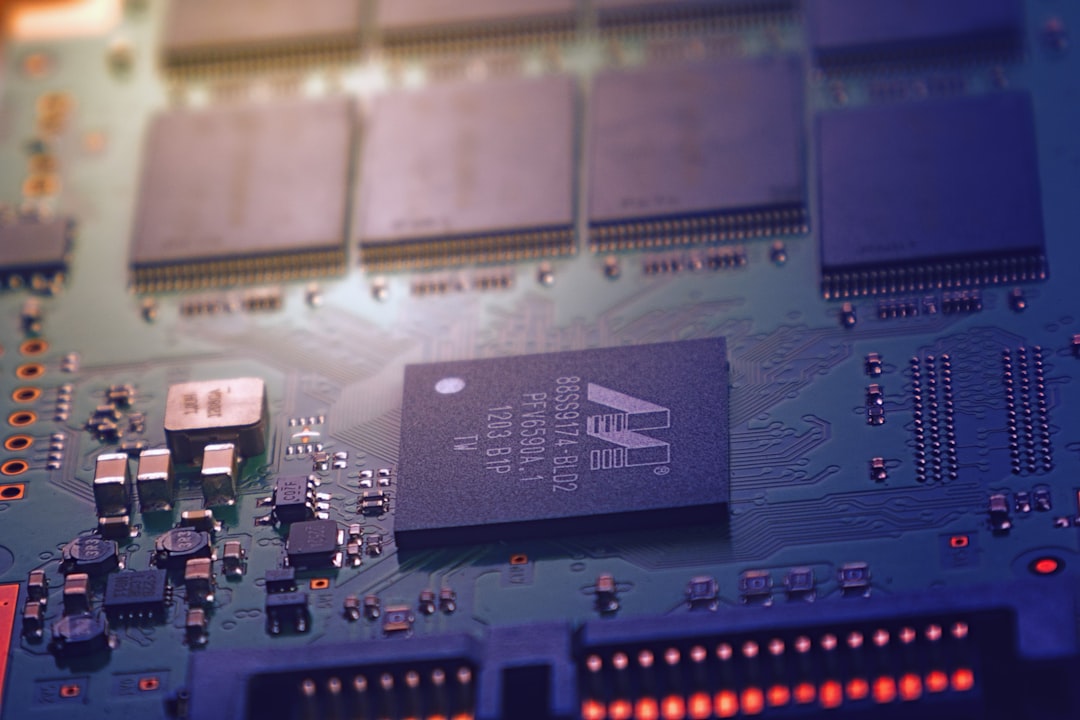
AI is transforming how color and light are adjusted in video post-production. These AI tools can automate a lot of the work that used to be done manually, like color grading, leading to faster editing workflows and more consistent results. The algorithms in these programs are getting better at analyzing video content and figuring out the best color adjustments on their own. This means editors can spend less time fiddling with settings to adapt to different lighting in a video. While the technology shows promise in terms of better video quality and faster turnaround times, we need to also be mindful of the potential downsides. Relying too much on automated processes could impact the creative choices and authenticity that give videos a unique feel. As AI continues to improve, it will likely present both exciting new opportunities and some challenges for editors, especially when it comes to artistic considerations in video editing.
The incorporation of AI into post-production is dramatically altering how we approach color and light adjustments in videos. These AI-powered tools can analyze the entire dynamic range of the footage and automatically suggest optimal enhancements, leading to a more natural representation of the original scene's depth. This often reduces the need for tedious manual grading, streamlining the post-production process.
Interestingly, some of these systems use color theory algorithms to adjust hue, saturation, and brightness, aiming to match the overall emotional tone of the video. This moves beyond just technical correction and aligns with the narrative intent of the content.
Furthermore, the temporal analysis capabilities of these systems allow for a consistent look across a video. They review consecutive frames to ensure smooth lighting transitions, which is crucial for fast-paced edits where changes between shots could be jarring.
One intriguing aspect is the use of machine learning to predict adjustments. AI-driven converters can leverage data from past projects to anticipate the required color and light corrections based on initial user settings. This predictive ability can greatly speed up the workflow by minimizing repetitive adjustments.
Advanced systems are beginning to use scene recognition technology. This means they can identify specific elements within a frame – like skin tones, landscapes, or urban environments – and then apply adjustments based on established visual norms for each element. This ensures every component of a scene is corrected according to common aesthetic standards.
Automatic adjustments commonly incorporate real-time histogram analysis. This allows the software to adapt the image's brightness and contrast based on the distribution of light, leading to more detail in both bright and dark areas, thus enhancing overall clarity.
It's also fascinating that these systems are becoming better at handling color gamuts. They can automatically identify and utilize various gamuts, like sRGB or Adobe RGB, making sure the video is optimized for the intended display platform. This is crucial for consistency between editing and final output.
Some advanced systems employ feedback loops to continuously learn from viewer reactions and engagement levels, constantly refining their algorithms over time. This creates a self-improving system that learns directly from viewer interaction, not just code updates.
Another benefit is that modern tools incorporate edge detection mechanisms to preserve important visual details while correcting other parts of the image. This helps prevent loss of detail in sharp edges, a common problem with traditional light adjustments.
While these automated systems offer a considerable boost in efficiency, it's crucial to acknowledge potential drawbacks. Over-reliance on them might lead to a homogenization of visuals, resulting in a subtle loss of unique artistic styles as projects start to look more standardized. The potential for artistic expression to be limited by an AI-driven approach requires careful consideration. As AI continues to evolve in post-production, striking the right balance between automation and artistic control will be an ongoing research area.
The Impact of AI on Online MP4 Video Converters Efficiency and Quality Improvements in 2024 - Deep learning techniques for video quality assessment
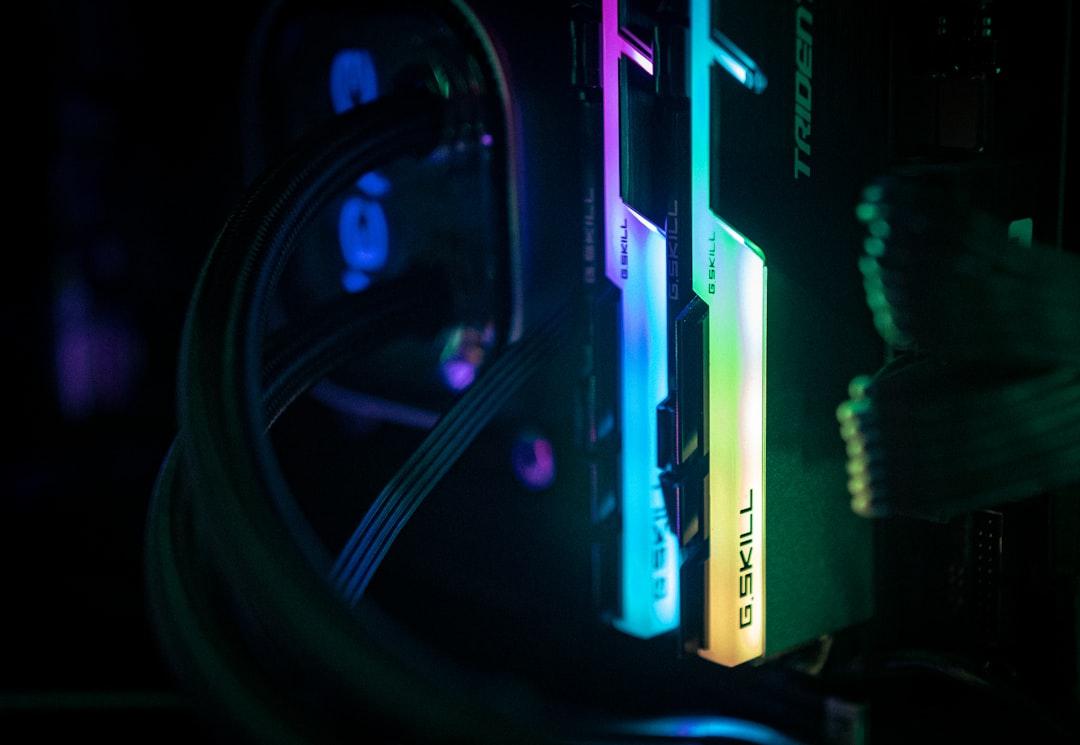
Deep learning is revolutionizing how we assess video quality, moving beyond simpler methods to capture the intricacies of human visual perception. These advanced techniques offer a more nuanced understanding of video quality by considering the trade-offs between efficiency and effectiveness. For instance, some new methods leverage deep learning to tackle challenges like linguistic ambiguity in image quality assessments. Furthermore, competitions like the AIS 2024 Video Quality Assessment Challenge are driving the development of new deep learning models that are specifically designed to handle user-generated content.
The push for more holistic approaches to VQA is seen in architectures like CNN-LSTM, which incorporate both spatial and temporal analyses to more accurately understand video imperfections. This move toward a more comprehensive understanding of video artifacts is a key trend in this space. As we continue to improve deep learning techniques for video quality, it's crucial to strike a balance between measuring technical quality with algorithms and gauging the actual viewer experience. It's an ongoing challenge to ensure that models align with how people truly perceive video quality.
Deep learning has become a powerful tool in video quality assessment (VQA), surpassing older methods that relied on simpler mathematical models. These newer approaches try to balance the effectiveness of the assessment with the computational cost. The CLIP-AGIQA method is a prime example of this, showing how a model like CLIP, originally meant for images, can be used for video quality assessment. It does have some limitations because of how language can be ambiguous.
Another interesting area is how AI is being used in video quality assessment for educational content. A recent study used linear models to analyze how well students accepted AI-generated video materials for language learning. The AIS 2024 Video Quality Assessment Challenge has been focusing on user-generated content (UGC) and encouraging people to come up with new deep learning methods for figuring out the perceived quality of videos from platforms like YouTube. It's worth noting that traditional video Quality-of-Experience (QoE) metrics often rely on the decoded video stream, but some are moving towards techniques that extract QoE scores from data available before or during video transmission.
Modular Blind Video Quality Assessment (BVQA) is important for video platforms because it lets you evaluate video content in subsampled formats without needing to know the exact spatial resolution or frame rate. It's critical for offering a good user experience, especially when videos need to adjust to different network speeds. CNN-LSTM architectures have been proposed to better evaluate video quality by simultaneously capturing spatial and temporal issues like artifacts.
One area of future research is how to use deep learning to assess the quality of a stream based only on server-side data—by incorporating network information along with the video itself. There's a huge need for methods to determine the perceptual quality of UGC videos because they can vary so much in terms of content and quality.
The current research in deep learning for video quality assessment points towards a future where models will be significantly more complex, capable of understanding the multifaceted nature of video imperfections and how people perceive them. It's still an active research area with plenty of challenges remaining before we have truly robust and universally applicable solutions for measuring video quality in all the various ways humans experience it.
The Impact of AI on Online MP4 Video Converters Efficiency and Quality Improvements in 2024 - Integration of 5G technology for higher-resolution formats
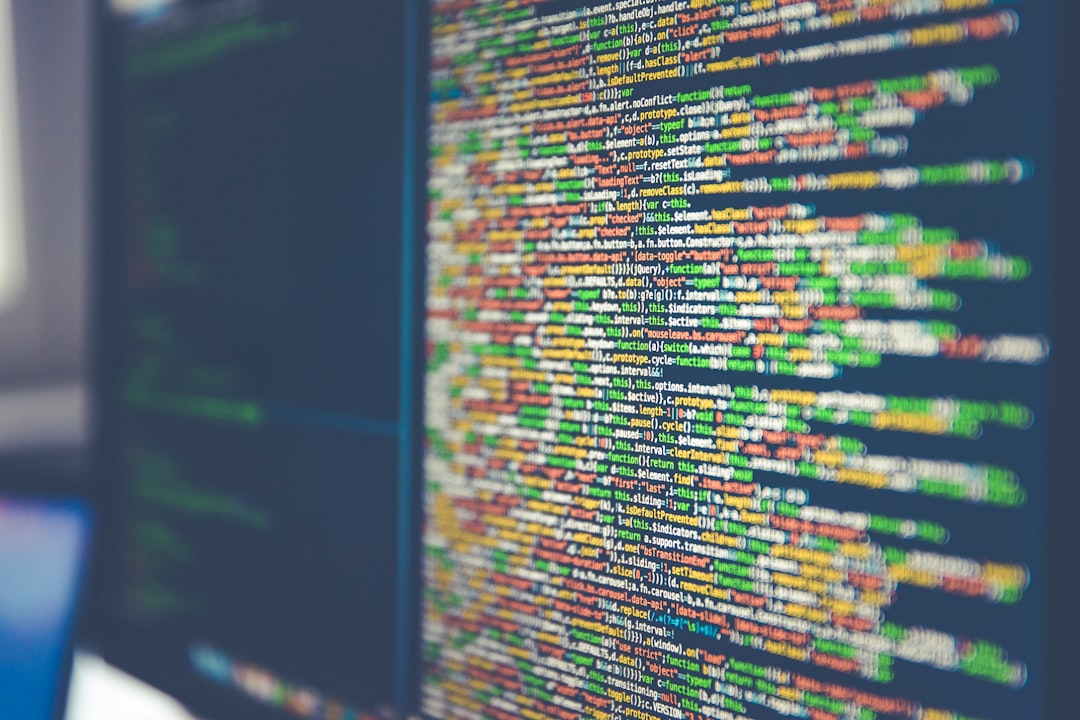
The rise of 5G is poised to transform how we experience high-resolution video formats. 5G's improved speed and capacity are crucial for delivering the massive amounts of data needed for 4K and even higher resolution videos, which require significantly more bandwidth than older formats. With 5G, the lag or buffering often associated with streaming high-quality video could be greatly reduced, leading to a smoother and more immersive viewing experience. Combining 5G's potential with AI's ability to adapt video quality to network conditions could further enhance efficiency, dynamically adjusting video quality to optimize bandwidth usage. However, these advancements also raise concerns about potential network congestion as more devices and users leverage 5G's capabilities. Additionally, the increase in data transmission raises important questions regarding data privacy and security in this increasingly connected world.
The integration of 5G with video formats is a fascinating development, particularly as it relates to higher resolutions. 5G's expanded bandwidth capacity is key here, allowing for smoother streaming of formats like 8K without the annoying buffering that can plague lower bandwidth connections. This is a significant step forward for content creators and consumers who desire a truly high-definition experience.
Furthermore, 5G's low latency capabilities are transformative. With latency as low as 1 millisecond, it opens up possibilities for real-time, high-resolution video interactions and streaming of events like esports or live sports, where immediate delivery of content is crucial. It's interesting to think about how this could change the way viewers interact with live events.
Beyond that, 5G's influence extends to edge computing. As these networks become more prevalent, edge computing is increasingly adopted for video processing. By pushing processing power closer to users, this strategy might help manage higher-resolution video streams more efficiently, relieving some of the burden on cloud-based systems. Whether edge computing ultimately becomes the dominant approach will depend on several factors, including cost and performance for different types of content.
Also, the combination of 5G and AI allows for dynamic resolution adjustments in video streaming. Algorithms can assess the capabilities of the viewer's device and network conditions and dynamically adapt the video stream to deliver the highest quality possible while minimizing bandwidth use. It's unclear how effective this will be in varied real-world situations, but it's definitely an interesting approach.
Moreover, this synergy between 5G and AI has the potential to revolutionize how videos are personalized. AI algorithms can learn from viewer preferences and behaviors, coupled with the higher data transfer rates of 5G, to deliver more tailored experiences. This ability to offer highly customized content, based on individual viewing habits, could become a powerful tool for engagement and satisfaction, though questions about user privacy and the potential for over-personalization remain.
The immersive experience aspect is another exciting area of 5G integration. With higher data transfer capabilities, we might see broader adoption of AR and VR technologies within video content. This potential to create incredibly detailed and interactive environments could completely change how we experience education, entertainment, and even training scenarios. How quickly this becomes mainstream depends on development of hardware and content creation methods.
Finally, the quality control aspect is also impacted by this convergence. AI algorithms, when integrated with 5G networks, can help monitor the quality of video streams in real time. They can identify and adjust for issues like pixelation and buffering, automatically adjusting to ensure a high-quality viewing experience, even under less-than-ideal network conditions. This could become a vital factor in providing consistent quality across varying network conditions.
The rapid advancements in 5G and AI technology, with their integration into video formats, presents an exciting landscape. We are likely to see continued development and optimization in the coming years as the technology matures. However, there's also a need to carefully monitor potential downsides like privacy issues and potential homogenization of content. Ultimately, striking a balance between innovation and its potential impacts will be a central theme in the future of video streaming.
The Impact of AI on Online MP4 Video Converters Efficiency and Quality Improvements in 2024 - AI-powered language localization for expanded audience reach
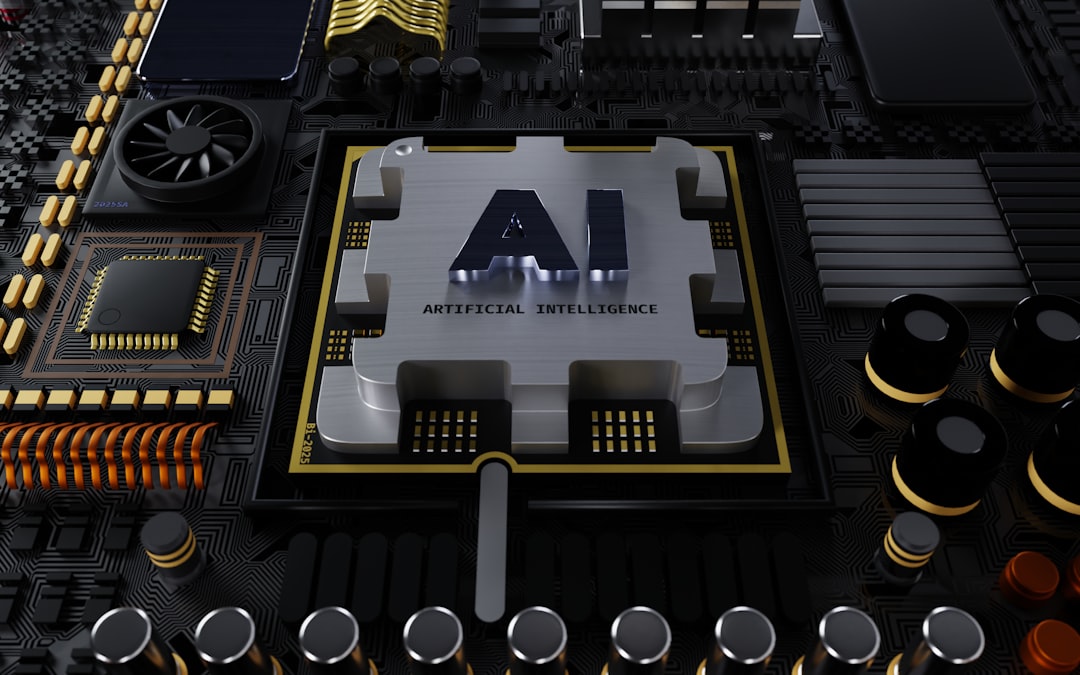
AI-powered language localization is transforming how content reaches wider audiences by providing more accurate and culturally-sensitive translations. This means businesses can more effectively engage people from different backgrounds, opening up new markets and growth opportunities. AI algorithms are designed to understand the nuances of language and culture, leading to translations that are not just technically correct, but also resonate with the intended audience. This improved accuracy can lead to a better overall experience for the viewer, as the content is more easily understood and appreciated. Furthermore, using AI for language localization can reduce the cost of translation, making it more accessible for smaller businesses or individuals looking to reach international audiences. While the technology continues to improve, it is showing promising results in 2024 and potentially could become more integrated with video production workflows, resulting in more engaging and globally accessible content. This ability to bridge language barriers through AI-driven adaptation has the potential to increase content consumption across borders, promoting wider understanding and inclusivity. However, it is important to remember that technology alone can't always capture all the complexities of human language and culture, and there will always be a role for human oversight and quality control to ensure that content is truly effective and avoids unintentionally creating misunderstandings or offense.
AI is progressively reshaping how videos are localized for wider audiences, leveraging sophisticated algorithms and machine learning to bridge language barriers and cultural nuances. While traditional localization relied heavily on human translators and often faced challenges with speed and accuracy, AI offers a new paradigm. It can analyze video content, including both visual and audio components, and generate translations that are contextually relevant and culturally appropriate.
For instance, AI can dynamically adapt not only subtitles but also audio commentary during video playback, ensuring viewers in diverse linguistic regions understand the content more readily. This real-time language adaptation is particularly useful for educational content, improving comprehension and viewer engagement. Furthermore, AI is increasingly capable of identifying cultural references and expressions, translating them in ways that resonate with local audiences, thus reducing misinterpretations and enhancing the overall relatability of content.
The efficiency of AI-powered translation is remarkable. Recent advancements showcase the ability to translate video subtitles significantly faster than human translators, sometimes in under 10% of the typical time required, with surprisingly high accuracy levels. This rapid translation capacity drastically streamlines the workflow for content distribution and enables faster market launches. Additionally, some AI tools analyze visual elements within the videos to enhance context awareness. For example, an AI system can differentiate branded clothing in the video and tailor translations accordingly, ensuring that the video's meaning is preserved across different language versions.
Moving beyond simply translation, AI is creating opportunities for greater personalization. Some algorithms can tailor language settings and even slang to user profiles and regional dialects, aiming for a more customized and engaging experience. This potentially leads to increased viewer satisfaction and retention. Furthermore, AI models can predict which languages will resonate with specific audiences in different regions, suggesting which translations would be most impactful before a video is even released, thereby increasing its potential for global engagement.
Interestingly, some AI localization solutions also include quality assurance mechanisms. These systems monitor viewer engagement metrics for various language versions and use this data to continuously refine their translation and adaptation processes. They can also adapt the video and subtitles to suit the formats of different platforms, optimizing the video for diverse environments, like social media, with little human intervention.
The potential for AI to reduce misinterpretations that arise from linguistic nuances and context is noteworthy. However, while this technology is promising, it's important to recognize that it relies on vast datasets and deep learning techniques, and its effectiveness can depend on the type of content and the complexity of the languages involved. Additionally, as AI-driven localization tools facilitate real-time collaboration among translators and editors, the overall localization process is expedited, leading to faster content releases.
Despite the advancements in AI-powered localization, we should remain attentive to possible limitations. It's crucial to ensure these tools strike a balance between automated efficiency and the preservation of a video's original meaning and cultural sensitivity. Nevertheless, AI's ability to address language barriers and cultural nuances, in combination with its speed and scalability, suggests a transformation in the accessibility of video content to global audiences. It's clear that this technology is steadily reshaping the landscape of video localization and will likely continue to refine and advance in the years to come.
More Posts from ai-videoupscale.com: