Upscale any video of any resolution to 4K with AI. (Get started for free)
Enhancing Video Quality How AI Upscaling Addresses Compression Artifacts
Enhancing Video Quality How AI Upscaling Addresses Compression Artifacts - AI-Powered Resolution Enhancement Techniques
AI-powered resolution enhancement techniques are transforming how we experience video. These techniques, fueled by sophisticated machine learning algorithms, significantly boost video quality by increasing resolution, minimizing noise, and refining fine details. Unlike older methods that simply fill in missing pixels through interpolation, AI approaches use neural networks trained on vast datasets to produce more accurate and visually pleasing results. This results in a more natural and engaging viewing experience, particularly for older video or content captured with lower-quality equipment. Leading-edge tools, such as NVIDIA's RTX platform and Adobe's VideoGigaGAN, showcase the capability of generating new visual elements, effectively enhancing the clarity of video that may have suffered from compression or age. As AI capabilities continue to grow, we can expect ever-more impressive levels of video quality improvement, potentially overcoming challenges related to compression artifacts that have plagued video for years.
AI-powered resolution enhancement techniques are increasingly leveraging convolutional neural networks (CNNs) for their ability to decipher the intricate patterns within images. These networks can effectively learn the spatial relationships present in images, which is especially helpful in recognizing and reconstructing the fine details often lost during video compression.
It's remarkable how these techniques can predict missing data. By examining patterns in high-quality images, the AI can intelligently deduce what the compressed image intends to convey, essentially filling in the missing visual information.
The recent advancements in generative adversarial networks (GANs) have led to a new generation of AI models that excel at producing high-resolution images. Not only do these models restore lost detail, but they also generate elements that blend harmoniously with the original content.
However, a major challenge in AI resolution enhancement is the potential for "over-smoothing." Excessive processing can result in overly uniform images, leading to the loss of important textures and a somewhat artificial, flat look.
One clear benefit of AI upscaling is the significant reduction in compression artifacts, like the distracting blocking and banding effects commonly observed in compressed video for streaming services. This leads to a much more pleasing visual experience, particularly on larger screens.
Unfortunately, the sophistication of these algorithms often comes at the cost of computational resources. Implementing them in real-time can be challenging unless you have robust hardware, making them less suitable for certain applications where immediate processing is crucial.
The performance of AI resolution enhancement is strongly influenced by the quality of the training data. AI algorithms need exposure to diverse and high-resolution images to develop effective upscaling techniques that can adapt well to new visual content.
It's worth noting that the application of resolution enhancement is not limited to videos; it also proves valuable for improving the quality of images captured from lower-resolution cameras. This benefit is especially significant in applications like surveillance and remote sensing, where detailed information is paramount.
While AI can dramatically enhance visual quality, it's essential to acknowledge the possibility of introducing new artifacts or distortions if the algorithms are not properly tuned or trained. This highlights the importance of carefully evaluating the fidelity of the output.
As the field continues to evolve, researchers are investigating potential applications in immersive environments like virtual and augmented reality. Here, high resolutions and minimal artifacts are essential for creating convincing experiences, expanding the potential impact of AI-powered resolution enhancement far beyond traditional video and image formats.
Enhancing Video Quality How AI Upscaling Addresses Compression Artifacts - Addressing Compression Artifacts Through Machine Learning

The increasing demand for high-quality video has highlighted the need for effective methods to address compression artifacts. These artifacts, which manifest as visual imperfections like blockiness and ringing, are often introduced during video compression to reduce file size and bandwidth usage. While traditional methods have attempted to address compression artifacts or resolution enhancement individually, machine learning, and especially deep learning, has opened up new avenues for a more comprehensive approach.
Deep learning models, such as ARCNN and DnCNN, are at the forefront of these efforts. By leveraging convolutional layers within neural networks, these models can effectively learn patterns in compressed video and reconstruct high-quality versions, simultaneously improving resolution and reducing artifacts. The ability to handle both aspects within a single framework represents a significant step forward.
However, challenges remain. The training of these networks relies heavily on the availability and quality of training data. Furthermore, careful tuning of model parameters is critical to avoid introducing unwanted artifacts or distortions during the artifact removal process. As the field advances, researchers are constantly refining algorithms and training methodologies to ensure that AI-based solutions provide the best possible video quality, ultimately leading to a more immersive and enjoyable viewing experience.
Compression artifacts, like the blocky patterns and color banding we see in compressed video, aren't just visual blemishes—they can actually change how we perceive the content. They can lead to misinterpretations of what's happening in a scene, highlighting the importance of tackling them effectively.
While traditional methods often struggle, machine learning models have shown remarkable promise in filling in missing pixel information by recognizing and recreating subtle textures. This ability to intelligently predict missing details offers a pathway to improved image quality.
One particularly interesting area is the use of generative adversarial networks (GANs). These AI systems use two neural networks that compete with each other, resulting in a refinement process that leads to better detail preservation. It's like a constant back-and-forth, pushing the output towards a more realistic outcome.
However, the story isn't always simple. The effectiveness of these AI methods often depends on the specific types of artifacts present. Some AI models are particularly good at reducing banding, while others excel at tackling blockiness. This suggests that a one-size-fits-all solution might not be ideal.
Research also suggests that training AI models on data that's specific to certain video genres or compression types can produce even better results. This idea of tailoring the training data for specific applications is a recurring theme in many machine learning fields and highlights the importance of selecting the right data for optimal performance.
While promising, there's a cautionary note: AI can sometimes introduce its own artifacts if not carefully calibrated. This underscores a constant tension in image processing—we're striving for enhancement while maintaining fidelity to the original content.
Intriguingly, many of the AI algorithms designed to improve video quality are built upon our understanding of how humans see. They integrate insights from vision science into their training process to generate outputs that appear more natural and believable.
Compression artifacts often lead to distorted color gradients, and there are AI techniques specifically designed to address this. By learning the optimal color distributions from high-quality source material, these algorithms can help restore a more natural color palette.
Processing video in real-time using AI is certainly feasible with optimized algorithms, but it usually requires specialized hardware like GPUs. This points to the need for further advancements in hardware to keep up with the rapid progress of AI software.
Finally, we should remember that even the most sophisticated AI techniques can only go so far. The success of these approaches depends heavily on the initial quality and resolution of the video. When dealing with heavily degraded source material, the ability of AI to make meaningful improvements might be limited.
Enhancing Video Quality How AI Upscaling Addresses Compression Artifacts - Comparing Traditional and AI Upscaling Methods
When examining how we enhance video quality, the differences between traditional and AI-based upscaling methods become apparent. Traditional methods, typically relying on interpolation, struggle to produce truly sharp and detailed results. They simply estimate missing pixels based on neighboring ones, often leading to a somewhat blurry or artificial appearance. AI upscaling takes a different route, employing machine learning to understand the underlying structure of images and video. AI algorithms can learn from a large collection of high-quality images, which enables them to intelligently fill in missing information and produce more realistic results. This approach offers significant advantages in addressing compression artifacts and enhancing details that traditional methods miss. However, this advance in image enhancement isn't without challenges. The quality of AI-based upscaling hinges heavily on the training data used to create the algorithm. Moreover, using these algorithms can be computationally demanding, requiring powerful processing capabilities. Despite these limitations, the potential of AI to significantly improve video quality and overcome common limitations of traditional methods is undeniable.
Traditional upscaling approaches often rely on straightforward techniques like bilinear or bicubic interpolation. While functional, these methods can sometimes lead to images that appear blurry or lack sharpness, especially when increasing resolution significantly. In contrast, AI upscaling employs machine learning models capable of recognizing more intricate patterns within the video data, often resulting in images that retain sharper details and textures.
The performance of machine learning models for video enhancement can vary greatly based on their design. CNNs, for example, have demonstrated a clear advantage over older models due to their proficiency in learning complex feature relationships across different parts of the image or video. This hierarchical understanding of image information makes CNNs particularly suitable for capturing fine detail.
One major shortcoming of standard upscaling is the difficulty in accurately preserving image textures during resolution increases. Textures, essential for giving a sense of surface detail and realism, are frequently lost or distorted using traditional methods. AI upscaling excels in identifying and reconstructing these textures, often leading to a more natural and visually appealing outcome.
It's quite fascinating that in some instances, AI-based video restoration techniques have shown the capability to outperform human video editors. This is largely due to their ability to analyze massive amounts of data and identify subtle improvements or restoration paths that might elude a human operator or even simpler algorithmic approaches. This ability to automatically process and analyze vast amounts of visual information is a powerful advantage that machine learning brings to the table.
While AI upscaling methods demonstrate clear strengths in mitigating compression artifacts, their effectiveness isn't uniform across different types of artifact. Some models excel at dealing with banding, while others are more adept at reducing blockiness. This contrasts with more generic traditional methods, where the approach remains largely constant regardless of the specific type of artifact.
The success of AI models in predicting and reconstructing missing visual information hinges strongly on the quality and variety of the data they are trained on. Interestingly, models exposed to a vast collection of diverse video content appear to generalize better when encountering new and unseen types of video. This broad training significantly contributes to their overall efficacy and applicability across diverse uses.
Real-time video processing using AI often demands considerable computational resources, primarily from powerful GPUs. This hardware dependence can make it impractical for some applications where immediate or low-power processing is crucial. Traditional methods, due to their simplicity, typically require significantly less processing power and are more readily executable on common consumer hardware.
AI upscaling methods show a remarkable capacity to adapt to specific video genres. By fine-tuning their training data, they can achieve impressive results tailored to specific genres like action, documentaries, or animation. This level of customization surpasses traditional methods, which generally employ fixed settings that don't account for diverse content variations.
It's important to acknowledge that even with their sophistication, AI upscaling can occasionally introduce new artifacts, such as over-smoothing or unwanted haloing effects. While less sophisticated, traditional methods sometimes produce more stable and predictable results due to the fewer assumptions they make about the input. This highlights the inherent trade-off between complexity and control.
Beyond video enhancement, AI upscaling exhibits exciting potential across a variety of other fields. Applications in medicine (medical imaging), earth observation (satellite imagery), and others depend on the precise reconstruction and interpretation of detailed information. These fields, where accuracy is paramount, present clear opportunities for AI upscaling to contribute where traditional methods might fall short.
Enhancing Video Quality How AI Upscaling Addresses Compression Artifacts - GANs Role in Reducing Video Distortion
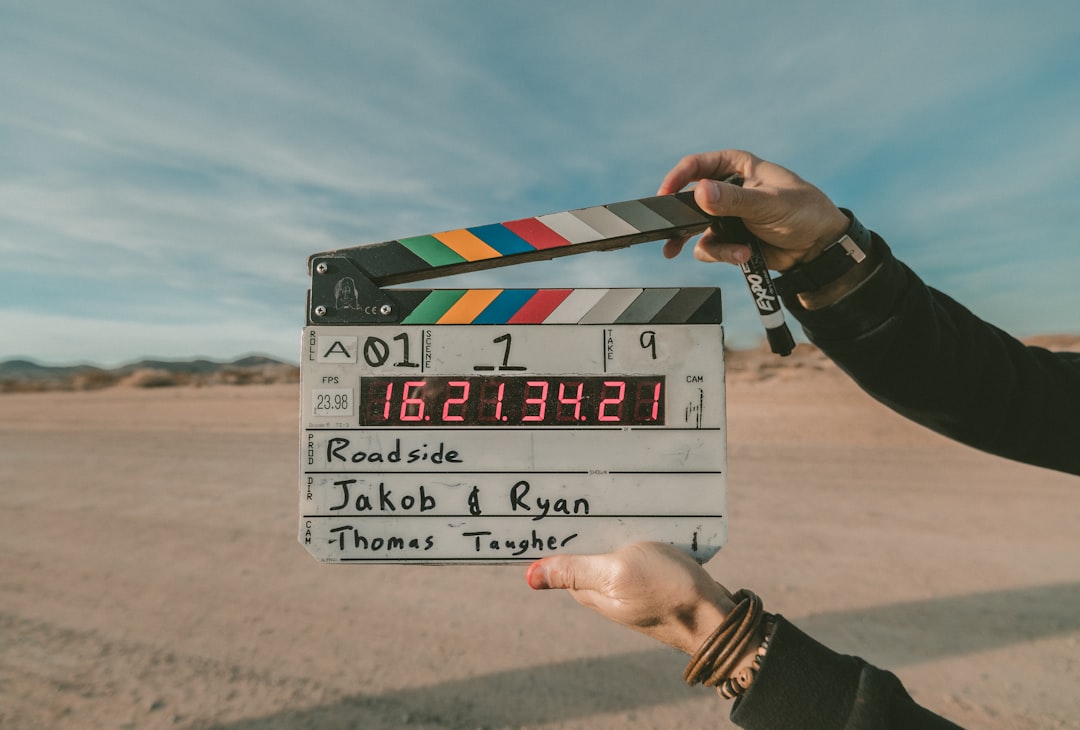
Generative Adversarial Networks (GANs) have emerged as a powerful tool in enhancing video quality, specifically in the task of reducing distortion caused by compression. These networks, particularly in models like SRGAN and ESRGAN, employ a unique adversarial training approach. This involves two neural networks competing against each other: one generates a higher-quality image from the compressed version, and the other evaluates the quality of the generated image against the original. This constant push and pull helps GANs refine their output, leading to a more accurate restoration of details that may have been lost during compression.
The effectiveness of GANs in minimizing artifacts like blockiness or color banding stems from their ability to learn and reconstruct subtle textures and patterns that are often overlooked by simpler methods. They strive to create a more perceptually realistic outcome, aiming for a higher level of visual fidelity compared to traditional methods which often rely on interpolation or other less sophisticated approaches.
However, this complex approach comes with its own set of difficulties. GANs can be computationally demanding, often requiring significant processing power, making real-time applications challenging without specialized hardware. Furthermore, the complex nature of the networks can lead to the introduction of new artifacts if not carefully managed.
Despite these issues, GANs are gaining significant traction in the field of video enhancement. As they continue to evolve, they are likely to play an increasingly important role in ensuring high-quality video experiences, especially as our reliance on online video streaming and communication grows. The ability to produce near-perfect high-resolution videos from lower-quality sources is an exciting frontier, and GANs are on the front lines of this pursuit.
Generative adversarial networks (GANs) employ a fascinating approach to video enhancement by using two neural networks that compete against each other. One network generates video frames, while the other acts as a critic, evaluating the quality of the generated frames. This constant back-and-forth drives the generator network to create increasingly realistic and detailed reconstructions, which is especially useful for restoring lost details in compressed video.
Unlike traditional methods that tend to focus on individual pixels, GANs work with a broader understanding of the scene. They learn patterns and relationships in the data, enabling them to fill in missing information in a more intelligent and contextually aware way. This leads to more natural-looking results that integrate seamlessly with the surrounding content.
Recent research suggests that GANs are surprisingly adept at learning from compressed video and extrapolating details that were lost during the compression process. This means they can recover subtle textures and color variations that might be missed by simpler techniques, ultimately enhancing the overall visual quality.
Furthermore, GANs demonstrate a unique ability to be trained specifically for certain types of compression artifacts. For instance, a GAN could be trained to tackle banding or blockiness more effectively than a more general-purpose upscaling algorithm. This targeted training allows for more precise and effective artifact reduction in specific scenarios.
The iterative nature of GAN training is another noteworthy characteristic. As the networks refine their abilities, the quality of the generated frames gradually improves, leading to a more refined and artifact-free output. It's somewhat akin to the fine-tuning that often takes place in manual video editing.
Interestingly, GANs can sometimes even surpass the quality of the training data in terms of detail and complexity. This is because they learn to blend different patterns and textures in creative ways, generating outputs that go beyond simply restoring the original content. This ability to introduce novel elements can enhance the viewer experience beyond simple restoration of degraded video.
However, GANs are not without their drawbacks. In certain cases, they can introduce undesirable artifacts like checkerboarding or ghosting, especially if the training process isn't carefully managed. This inconsistency in output quality raises questions about the reliability and predictability of GANs in various applications.
Real-time video processing using GANs remains a considerable challenge due to the high computational demands of the dual-network architecture. It necessitates sophisticated parallel processing techniques, which can be difficult to implement on common hardware. This represents a significant hurdle for GANs in applications that require immediate processing, like live video streaming.
A compelling aspect of GANs is the ability to integrate user-defined parameters into the generation process. This allows for customization of the restoration process, such as controlling the degree of detail or even adding an artistic touch to the output. This level of control surpasses the capabilities of more traditional methods, which often provide a fixed set of options for upscaling.
The exploration of GANs for frame interpolation in video is an exciting area of ongoing research. In frame interpolation, the GAN generates new frames to fill in gaps between existing ones, resulting in smoother playback. This application not only reduces the visibility of compression artifacts but also holds potential for enhancing cinematic experiences by challenging traditional frame rates and offering new forms of visual storytelling.
Enhancing Video Quality How AI Upscaling Addresses Compression Artifacts - Real-Time AI Processing for Streaming Platforms
Real-time AI processing is transforming how streaming platforms deliver video. These platforms are increasingly using AI-powered upscaling to tackle common compression issues that result in blocky or blurry videos. The use of advanced algorithms, including NVIDIA's RTX Video Super Resolution and Adobe's VideoGigaGAN, shows that the generation of new visual elements is possible, offering a considerable improvement over simply duplicating existing pixels. These AI models can significantly improve sharpness and clarity by filling in details lost during compression, offering a much more refined and immersive viewing experience. This advancement isn't limited to high-powered computers; real-time upscaling is becoming feasible even on mobile devices. However, AI upscaling is computationally intensive and often requires powerful hardware, making it challenging for certain devices and applications. The ongoing trade-off between improved visual quality and necessary hardware remains a key challenge in achieving consistently high-quality video streaming across the various platforms and devices people use today.
AI is increasingly being used to process video in real-time, especially within streaming platforms. Machine learning algorithms can now handle frame-rate conversions up to 120 frames per second (FPS), which is particularly helpful for smooth playback of fast-paced action scenes without losing quality. It's fascinating how AI can analyze motion and predict the flow between frames, generating intermediate ones to ensure a seamless viewing experience even in very dynamic scenes.
Furthermore, AI is capable of doing things like background removal in real time. This could be a great benefit to live streamers who want to create a more focused, less cluttered experience for their viewers. These algorithms are also getting good at dynamically adjusting bandwidth, which is clever because they can automatically choose the best quality based on the current network conditions. This means viewers are less likely to experience interruptions or buffering, even if their internet connection isn't top-notch.
Interestingly, there's a significant potential for AI to decrease latency in streaming. Some studies have shown latency reductions of up to 30%. This would make a big difference in things like live sports, where the time between an event and seeing it on a stream is important for a good experience. AI is also moving into the realm of audience analysis and customization. AI models can track things like viewer engagement and preferences, which then allows for on-the-fly adjustments to settings like resolution, frame rates, and bit rates.
Beyond these aspects, AI is also showing potential for improving color accuracy. Algorithms are being developed to analyze the color distribution of frames and make adjustments in real-time. This enhances visual consistency and fidelity during streaming, regardless of the conditions. It's also worth noting that real-time AI is being integrated with edge computing, moving some of the processing closer to the viewer's device. This reduces the load on central servers and improves responsiveness for users.
We're even seeing AI handle older video formats by performing deinterlacing in real time. This improves the quality of content from older sources while still preserving the vintage feel, which can be important for classic movies or shows. One final point about the efficiency of AI is that it can reduce data usage by up to 50% during streaming through better compression techniques. This is significant, as it can allow users to stream high-quality video even in areas with limited data access or slow connections.
While many of these advancements are quite promising, it is important to consider that some of these AI systems require specialized hardware to run at the speeds and with the capacity needed for real-time performance. Also, the performance is directly related to the quality of the training data, so it's very important to understand how AI training data is collected and validated. There is always a trade off to consider, it can be both exciting and daunting to see these systems advance.
Enhancing Video Quality How AI Upscaling Addresses Compression Artifacts - Future Developments in AI Video Enhancement Technology
The future of AI video enhancement holds the potential for a significantly enhanced viewing experience. We can expect advancements in AI algorithms, particularly GANs, to provide a deeper understanding of video artifacts, leading to more precise and targeted solutions for reducing issues like compression-related blockiness and color banding. Furthermore, real-time AI processing is poised to become more widespread, enabling a wider range of devices and applications to benefit from AI-powered upscaling, even those with limited processing power. However, the inherent complexity and high computational demands of these advanced algorithms pose a continuing challenge. Future progress will require innovations in both algorithm design and hardware performance to ensure that AI-driven enhancements are seamless and readily accessible. Maintaining a balance between video quality enhancement and preserving the integrity of the original source material will remain a critical factor. Excessive reliance on AI could unintentionally introduce new artifacts or distortions, so the development of these technologies must be carefully managed to avoid such unwanted side-effects.
AI video enhancement technology continues to evolve, with researchers exploring intriguing avenues for improvement. One fascinating development is the ability of some AI models to subjectively enhance video quality beyond the original source material. By blending details learned from a wide range of high-quality videos, they can produce outputs that are visually more appealing than the original, even if technically not a "higher fidelity" restoration.
There's a growing trend towards "cross-domain learning", where AI models trained on one video genre can be effectively adapted to other, quite different, genres. This approach is incredibly useful for improving training efficiency while still maintaining high performance. However, challenges remain, particularly in maintaining temporal coherence. Many AI-based methods excel at enhancing spatial detail but sometimes struggle with frame-to-frame consistency in dynamic scenes, especially during fast cuts, which can negatively impact the viewing experience.
Despite their impressive capabilities, even advanced AI models can inadvertently introduce new artifacts, such as 'ghosting' or 'checkerboarding'. While these added details may seem plausible, they might not align with the physical properties of the scene, raising concerns about the reliability of these systems.
Excitingly, researchers are creating more adaptive AI frameworks that can respond to changing compression artifacts and content types in real time. These models learn from incoming video streams and potentially viewer preferences, allowing them to optimize video quality dynamically for each individual viewing experience. The efficacy of these adaptive frameworks, however, is still tied to the diversity of the data used during training. AI models that are trained on a wider array of compression types tend to generalize better when faced with new, unseen videos.
Some AI models are incorporating principles of human visual perception into their design. This means they try to improve the experience of watching a video by understanding the visual cues humans find important, rather than just focusing on metrics like resolution or pixel count. There's also a growing focus on designing specific AI solutions for various video compression methods (like H.264 vs. VP9). This targeted approach allows for the effective recovery of unique artifacts specific to each algorithm, as opposed to applying one universal solution.
Furthermore, we see a shift towards integrating real-time feedback loops. This means viewers' interactions can directly inform future video processing by the AI. As viewers watch, their engagement patterns can tell the AI what works and what doesn't, creating a dynamic environment for enhancement.
Finally, the realm of AI-powered frame interpolation is opening new possibilities. Generative models can predict and create new frames to fill gaps between existing ones, resulting in smoother playback at higher frame rates. This not only creates a more enjoyable viewing experience but also reduces the noticeable impact of compression artifacts. While this field is very promising, it still faces challenges with its computational demands.
In conclusion, AI video enhancement technology has the potential to fundamentally reshape how we interact with video, pushing the boundaries of visual fidelity and creating more immersive experiences. While the journey is ongoing, and hurdles like maintaining temporal coherence and mitigating unwanted artifacts persist, ongoing research continues to address these concerns. Ultimately, AI's ability to adapt, learn, and integrate human perception promises a future where video quality continuously improves and aligns with the changing needs and preferences of the viewer.
Upscale any video of any resolution to 4K with AI. (Get started for free)
More Posts from ai-videoupscale.com: