Efficient Batch Conversion A Guide to Transforming Multiple Video Formats Using AI Upscaling Tools
Efficient Batch Conversion A Guide to Transforming Multiple Video Formats Using AI Upscaling Tools - Understanding AI-Powered Video Upscaling Technology
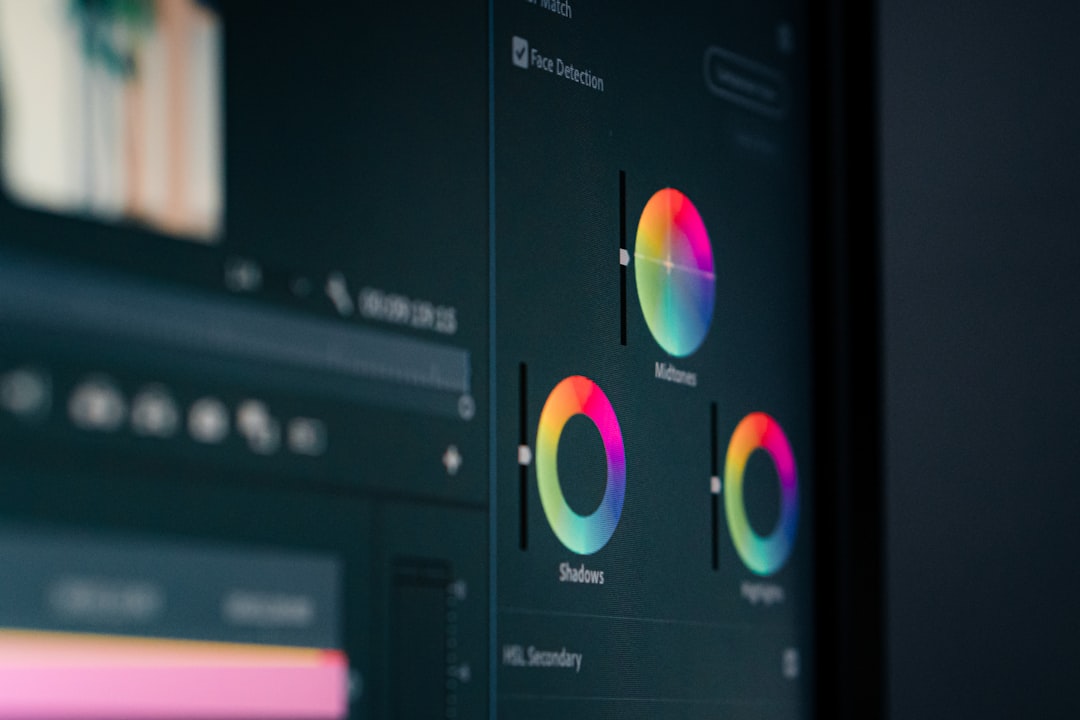
AI-powered video upscaling is a powerful tool for anyone who wants to improve the quality of their digital content. It can be used to turn blurry, low-resolution videos into crystal-clear high-definition ones, making older footage look almost new. The process involves using sophisticated algorithms that analyze each frame of the video and then fill in the missing details to create a more realistic image. This is particularly useful for home videos and archival footage, which often suffer from poor resolution and other imperfections.
While AI upscaling offers many benefits, it's important to recognize that it's not a magic bullet. The quality of the output depends heavily on the quality of the original video, and even the most advanced algorithms can only do so much. As the technology continues to evolve, however, we can expect even more impressive results in the future, with tools capable of achieving even higher levels of detail and realism.
AI video upscaling technology has become increasingly sophisticated, pushing the boundaries of video quality enhancement. It primarily relies on convolutional neural networks (CNNs), which are trained on massive datasets of images and videos to learn intricate patterns. This allows them to generate more realistic and convincing results than traditional upscaling methods.
Many upscaling algorithms utilize a technique called "super-resolution," where multiple lower-resolution frames are combined to create a single high-definition output. This process effectively reduces noise and artifacts while enhancing the overall clarity.
However, the effectiveness of AI-powered upscaling varies depending on the type of content. Animated videos might respond differently than live-action footage because of their distinct textures and details. It's important to consider the unique characteristics of the video when applying these techniques.
Upscaling tools often utilize extensive training datasets comprised of high-quality images and videos, with some models incorporating billions of parameters. This enables them to make nuanced transformations that are more context-aware and capable of mimicking the subtle intricacies of real-world footage.
While impressive results can be achieved, there are challenges. Algorithms may struggle with certain types of motion, resulting in artifacts like ghosting or blurriness. This highlights the ongoing need for research and improvement in AI upscaling techniques.
The future of video upscaling may involve multi-modal machine learning, where information from both audio and visual inputs is combined to produce more coherent and contextually aware enhancements. This field is still in its early stages, but it holds great potential for creating truly immersive and realistic experiences.
Efficient Batch Conversion A Guide to Transforming Multiple Video Formats Using AI Upscaling Tools - Selecting the Right AI Tool for Batch Video Conversion
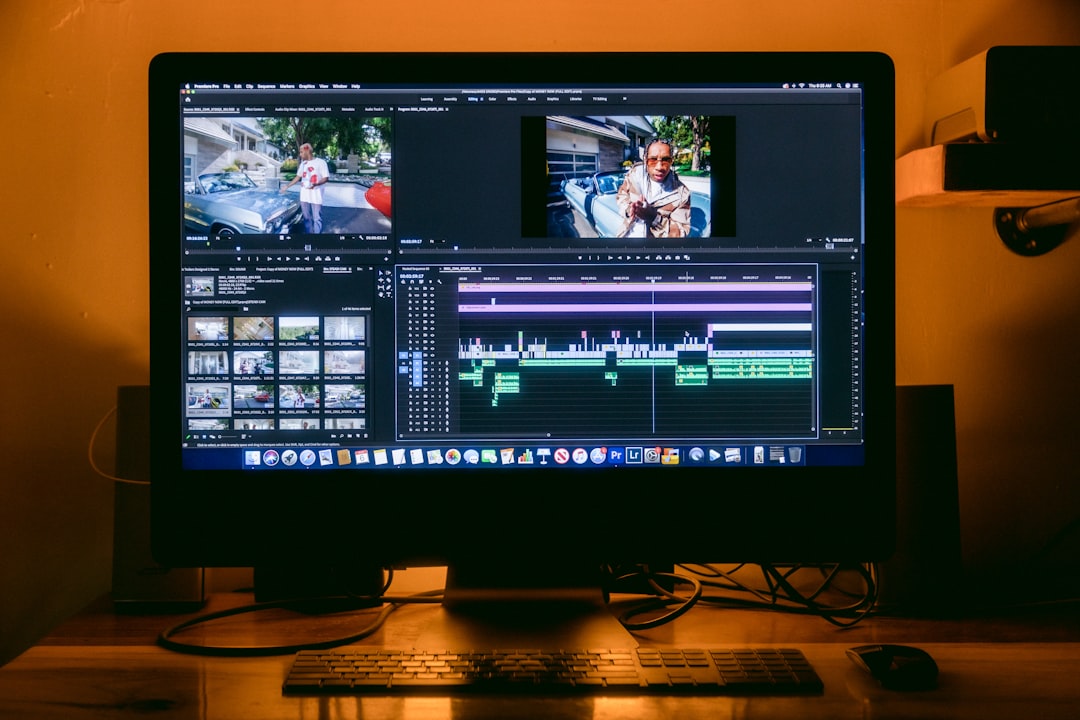
Choosing the right AI tool for batch video conversion is crucial, as it can significantly impact your overall workflow and the quality of your final product.
You need to carefully consider several factors when making your selection. Look for a tool with a user-friendly interface that allows you to easily manage and process multiple videos. The tool should also process efficiently and maintain high output quality, ensuring that your upscaled videos look sharp and natural.
While there are various options available, some popular choices include VideoProc Converter AI and Topaz Video Enhance AI. These programs offer advanced upscaling capabilities and batch processing features that cater to both beginners and professionals.
It's important to determine whether you prefer free or paid versions, as well as the available subscription plans. Your budget and specific requirements should drive your decision. Ultimately, the best tool for you will depend on the characteristics of your video content and the level of enhancement you want to achieve.
Selecting the right AI tool for batch video conversion can be a challenge, as many factors influence the final result. It's not just about finding a tool with a fancy interface or claiming super-fast speeds. Here's what you should consider:
1. **Codecs:** Check for compatibility. A tool that supports your video's codec will make the process much smoother and quicker. This is an often overlooked aspect, but it can make a big difference in how your video converts.
2. **Hardware Matters:** The performance of an AI tool is heavily influenced by your computer's hardware, especially the graphics card (GPU). Some tools are optimized for specific GPU manufacturers (NVIDIA, AMD), so using a different one may not give you the best results.
3. **Resolution Limits:** Be aware of how much resolution an AI tool can handle. If you're working with older footage or want to upscale to high resolution, you'll need a tool that can keep up. Some may be limited to Full HD, while others can go beyond.
4. **Training Data Biases:** The effectiveness of an AI tool is also connected to the quality and variety of the data it was trained on. This can mean a tool might be better suited for certain types of video (like live action or animation). It's a reminder that AI is still learning, and biases in training can influence results.
5. **Actual Processing Times:** Don't rely solely on advertised speeds. In real-world use, complex videos or high-resolution content may take longer than expected. It's good to test tools with your own video types before making a commitment.
6. **Dealing With Artifacts:** Different AI tools have different approaches to reducing unwanted artifacts like ghosting or blur. Some use more complex filtering, while others may introduce additional steps that could affect the quality.
7. **Control Over the Conversion:** Some tools offer adjustable settings that let you fine-tune the process to your liking, for example, setting how much noise reduction to apply or how strong the enhancement should be. This can be helpful in getting the best results for specific video types.
8. **Workflow Integration:** The tool you choose should integrate smoothly into your existing workflow. Look at whether it plays nicely with your video editing software or other tools you use. Having a seamless experience saves time and effort.
9. **Don't Just Go For The Expensive Option:** Price doesn't always equal quality. You might find great free or budget-friendly options that suit your needs just as well as high-priced tools.
10. **Multitasking Considerations:** How the tool performs when you're working on multiple video conversions at the same time is also a factor to consider. Some tools handle this well, while others may struggle and produce less quality outputs.
As a researcher and engineer, I find this a fascinating area with much room for improvement. It's great that AI tools are constantly being developed and evolving, but remember: the best tool is the one that meets your specific needs and delivers the desired results. Always do your own research and testing to make the best choice for your projects.
Efficient Batch Conversion A Guide to Transforming Multiple Video Formats Using AI Upscaling Tools - Preparing Your Video Files for Efficient Processing
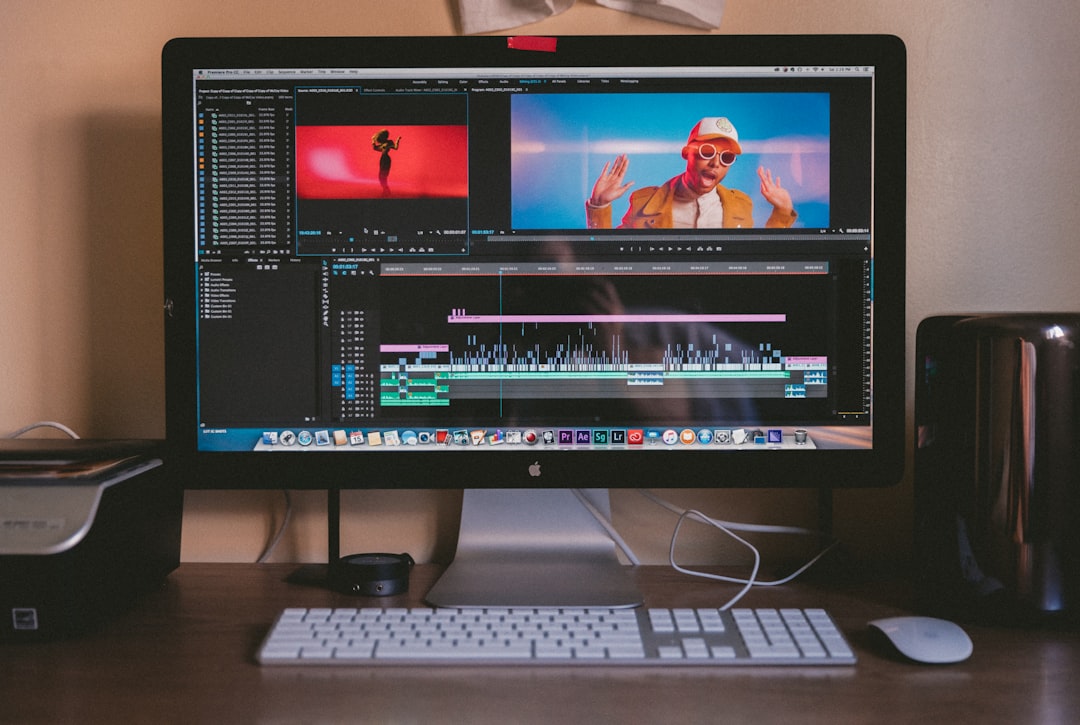
Preparing your video files before you begin batch processing is essential for a smooth and efficient conversion. This step often gets overlooked, but it can make a big difference in how your conversions go. The first thing to think about is the format of your video files. MP4 is generally considered a good choice because it compresses video effectively while still maintaining quality. Tools like FFmpeg or Handbrake can be helpful for converting files into MP4, especially if you're working with many videos at once. You'll also want to consider using software that takes advantage of your computer's hardware (like your graphics card) for faster processing. This is especially helpful when dealing with large video datasets. Basically, the better prepared your files are, the faster and smoother the conversion process will be.
As a researcher and engineer, I'm always looking for ways to make video processing more efficient, especially when dealing with large batches of files. While AI-powered upscaling offers a great way to improve video quality, it's important to remember that the process itself relies heavily on the source material. Preparing your video files carefully can dramatically impact the efficiency and quality of the results.
It's not just about the video format. Different codecs have different levels of compression, which can influence processing times. High bitrate videos offer great quality but also demand more computational power, potentially slowing down your batch conversion. Audio plays a role too. While we focus on video, some tools might spend significant time on audio processing, which can also impact efficiency, especially if you have high-fidelity audio tracks.
The source video itself matters too. Unsteady or shaky footage can cause problems for the upscaling algorithms, leading to unwanted artifacts. Ideally, you want a stable, solid source for the best results. Keyframes, those anchor points within a video that guide its motion, can also affect processing speed. More frequent keyframes help algorithms work faster, while fewer keyframes can lead to increased complexity and slower conversion times.
Remember, dynamic range is crucial. Videos with limited dynamic range can make it difficult for algorithms to enhance contrast and details effectively. For the most part, we want consistent material. Frequent cuts or sudden changes can confuse algorithms, potentially leading to inconsistencies in the upscaled output.
Keep in mind that AI upscaling tools are trained on specific datasets. A tool designed for cinematic content might not perform as well on amateur footage due to differences in quality, lighting, and color grading. It's important to choose the right tool for the type of videos you're working with.
And let's not forget about compression. If your video file has already been heavily compressed, there's a chance it will introduce noise that AI tools struggle to remove, which could limit the final enhancement outcomes.
Lastly, the number of videos you process in a batch can affect performance. Larger batches can overwhelm CPUs and GPUs, which can lead to slower processing speeds and potentially lower-quality output.
It's all about optimizing the input for the most efficient and effective AI-powered video upscaling. While the technology is amazing, we need to make sure we're feeding it the right data for the best possible results.
Efficient Batch Conversion A Guide to Transforming Multiple Video Formats Using AI Upscaling Tools - Setting Up Optimal Conversion Parameters

Setting up optimal conversion parameters is a vital step in any video batch conversion process. It's like finely tuning an engine for peak performance. You'll need to choose the right tools for the job, whether it's Handbrake, FFmpeg, or another option. Each one has its own strengths and quirks. For example, FFmpeg is known for its command-line power, while Handbrake is more user-friendly. You'll also want to consider how to handle audio settings. The best tools for the task can sometimes add unwanted noise if you aren't careful. The goal is to streamline the process by choosing a consistent format and codec, managing output paths, and using commands like "cv copy" in FFmpeg when appropriate. Remember, a little planning goes a long way toward getting the most out of AI upscaling tools and producing the best quality results.
Setting up optimal conversion parameters is crucial for efficient batch video processing, and I'm constantly intrigued by how AI tools are evolving to handle this complex task. While AI upscaling can greatly improve video quality, it's not just about throwing videos into a tool and hoping for the best. A lot of factors influence how well these algorithms perform.
One interesting development is dynamic processing. Instead of using a static set of rules, some AI tools adjust their approach based on real-time feedback about your hardware, the type of video, and even the progress of the conversion itself. It's like having a smarter, more adaptable tool that can make decisions on the fly to optimize performance.
Another area of advancement is frame interpolation. This technique allows tools to create new frames in between existing ones, which can smooth out jerky motion or even boost the frame rate of a video. It's a neat trick that opens up possibilities for enhancing older footage or making animations look smoother.
But it's not all sunshine and roses. Different types of artifacts, like pixelation or those annoying halos around edges, respond differently to AI algorithms. This means it's not a one-size-fits-all approach, and sometimes you might get results with certain artifacts still present. This emphasizes the need for a deeper understanding of how these algorithms work to achieve the best outcomes.
The same goes for things like video motion. Rapidly changing scenes or shaky footage can challenge algorithms, leading to inconsistencies in the final output. So, it's important to consider how stable your source footage is, as this directly impacts the effectiveness of the upscaling process.
GPU optimization is another factor to consider. Some AI tools are specifically tuned to work with particular graphics cards, so the performance might vary based on your hardware. Choosing a tool that's optimized for your GPU can lead to faster conversions and higher-quality results.
As a researcher, I'm always on the lookout for new ways to optimize video processing. It's exciting to see AI tools evolving, but it's also important to be aware of their limitations. Understanding the factors that can influence efficiency and quality will help me make better choices for my video processing projects.
Efficient Batch Conversion A Guide to Transforming Multiple Video Formats Using AI Upscaling Tools - Maximizing GPU Utilization for Faster Batch Processing
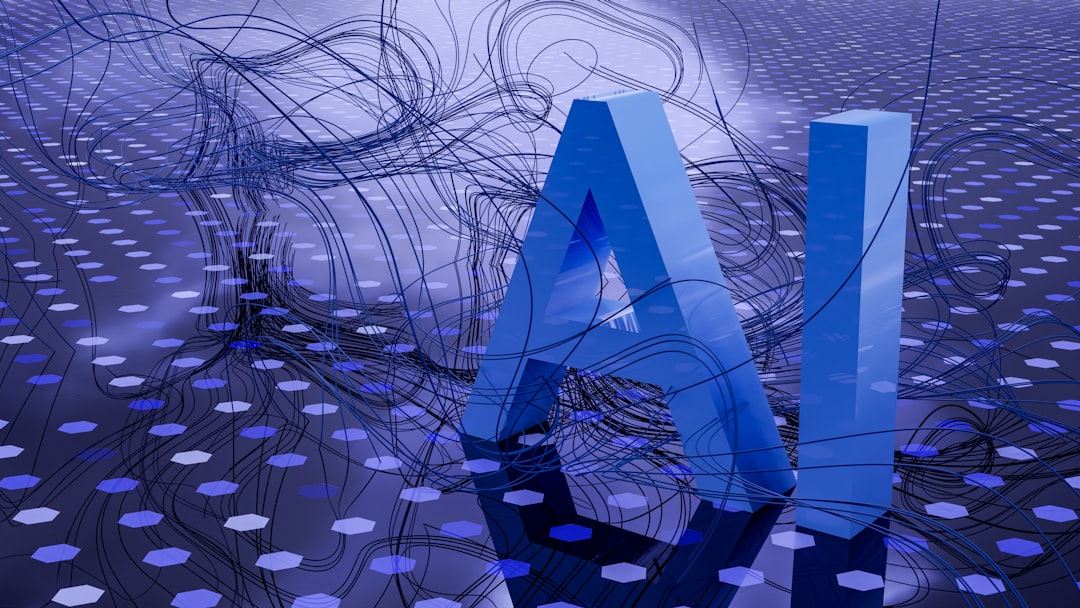
Maximizing GPU utilization is key to speeding up batch processing, especially when using AI for upscaling videos. Larger batches are great for utilizing the parallel power of GPUs, but you need to watch out for memory limitations and how it might affect the accuracy of the final results. Using tools like CUDA helps speed things up, but you'll want to keep an eye on how different batch sizes affect performance and fine-tune it to get the most out of your GPU.
Maximizing GPU utilization for faster batch processing is crucial for efficient video upscaling. GPUs are specifically designed for parallel processing, making them ideal for handling the heavy computational demands of AI-powered upscaling. However, understanding how GPU architecture affects performance is vital. NVIDIA's Ampere and Turing architectures, for instance, leverage Ray Tracing Cores and Tensor Cores, boosting performance for AI tasks like upscaling. This translates to faster processing times for our batch conversions.
High data bandwidth is another critical factor. Modern GPUs can move data at incredibly fast speeds, often exceeding 1 TB/s. This enables them to handle large video files and complex operations with ease. The parallel processing capabilities of GPUs are essential here. Their thousands of smaller cores allow them to handle multiple video frames concurrently, resulting in significantly faster processing compared to traditional CPUs that work sequentially.
Interestingly, GPU architectures also support varying precision levels in calculations, such as FP16 (half precision) and FP32 (single precision). Leveraging lower precision for certain tasks can lead to significant speed improvements without noticeably impacting output quality. It's a balancing act that demands a nuanced approach.
Efficient memory management is another vital aspect of GPU performance. Strategies like memory pooling and data locality can minimize latency and boost processing speeds. This is especially crucial when working with high-resolution video files, which require significant memory allocation.
Monitoring GPU utilization metrics during processing provides valuable insights into the efficiency of our workflow. Ideally, a well-optimized batch process should keep GPU utilization above 80%, indicating maximum efficiency. Anything lower might suggest bottlenecks that need to be addressed.
Algorithm optimization also plays a significant role. Tailoring upscaling algorithms to the specific GPU architecture used can yield remarkable performance gains. These optimized algorithms can reduce processing times by up to 50%.
While tempting, overclocking can increase processing speed by boosting the GPU's clock speed beyond the manufacturer's specifications. However, it comes with risks such as increased heat generation and potential hardware failure. It's a delicate balance between performance gains and potential risks.
Load balancing across multiple GPUs is another technique that can dramatically increase throughput and reduce conversion times. Distributing tasks across multiple GPUs allows for greater parallel processing, which is especially beneficial for larger video sets.
Dynamic resolution scaling is a fascinating development in video upscaling. Some advanced algorithms dynamically adjust resolutions based on GPU load and available memory, ensuring that the GPU operates at peak efficiency without compromising output quality. It's a clever approach that adapts to changing conditions for optimal performance.
As researchers and engineers, we constantly explore new ways to optimize video processing. Understanding how GPU architecture, data bandwidth, parallel processing, precision, memory management, algorithm optimization, load balancing, and dynamic resolution scaling all work together is essential for maximizing efficiency and achieving the best results in batch video processing.
Efficient Batch Conversion A Guide to Transforming Multiple Video Formats Using AI Upscaling Tools - Managing Output Quality and File Size Trade-offs
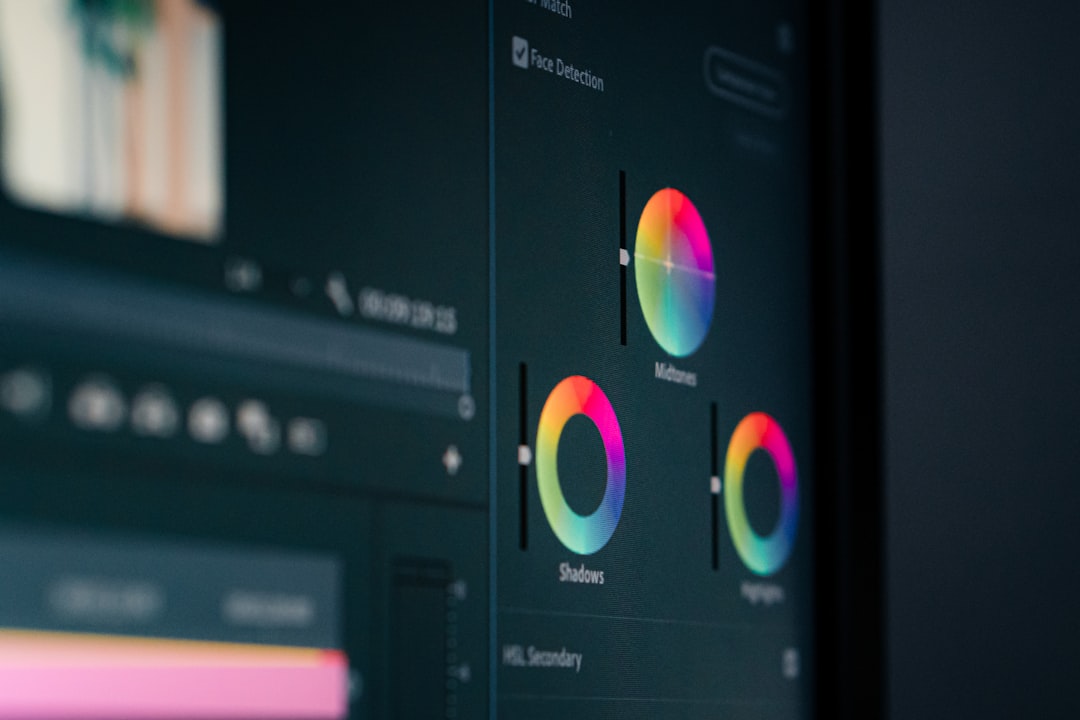
Balancing output quality with file size in video conversion is a tightrope walk. When dealing with batches, getting this right impacts efficiency tremendously. You need to find that sweet spot between clarity and file size. It's about optimizing things like how many files you convert in one go (batch size) and how the video is encoded. Smaller batches might mean better-looking videos, but they take longer and use up more resources. On the other hand, larger batches speed things up but could lead to a less detailed outcome if not handled correctly. Finding the best mix of batch sizes for making your videos and getting them ready for sharing is key for any video project.
Balancing output quality and file size is a constant dance when working with video compression. Even subtle tweaks to parameters like bitrate can dramatically affect the final product, often with a logarithmic relationship. A 10% drop in bitrate, for example, can cause noticeable decreases in clarity, leading to banding in gradients. This is especially important when dealing with high resolutions, like 4K. While these resolutions deliver stunning detail, they can inflate file sizes up to four times compared to 1080p, making bandwidth a major concern for many applications.
Compression algorithms themselves contribute to this dilemma. H.264 is a champion for efficiently handling moving images, while H.265 achieves similar quality with smaller file sizes, but requires more computational power to decode. This suggests that content type significantly impacts the choice of compression algorithms, making it more of a tailored approach than a universal solution.
The human eye's perception of color versus brightness is further explored in video compression using psycho-visual models. By adjusting the resolution in areas that are less visually salient, we can reduce file sizes with little to no perceived quality loss.
Batch processing speed is another factor. Simple scenes often process up to 30% faster than highly dynamic scenes because they require less motion compensation during compression, highlighting the influence of content complexity on efficiency.
Fortunately, some AI upscaling tools are starting to employ dynamic bitrate adjustment, changing compression rates based on the complexity of individual frames. This clever tactic optimizes quality and reduces file size without sacrificing the overall viewing experience.
Beyond compression, metadata and file sizes are closely tied. Unnecessary tags or high-resolution thumbnails can significantly increase file sizes, sometimes accounting for 5-10% of the total. This underscores the importance of metadata management in optimizing storage efficiency.
Color depth, another important consideration, can also significantly impact file size. Reducing color depth from 10-bit to 8-bit may shrink the file by up to 30% but can introduce banding artifacts, especially in gradients. This trade-off becomes a critical factor in maintaining visual fidelity when working with compressed formats.
Variable rate encoding (VBR), often used in modern encoding techniques, offers a balanced approach to quality and file size. It reduces file sizes during less complex scenes while maintaining quality in high-motion sequences, a useful strategy when seeking a good balance.
On the horizon are compression standards like AV1, promising up to 50% more efficient compression than HEVC (H.265). This potential to achieve smaller file sizes for similar quality, however, comes with a cost - increased computational demands during encoding and decoding, which could impact batch processing performance.
Navigating this complex web of trade-offs requires careful analysis and understanding the tools at hand. Balancing output quality, file size, processing speed, and other factors is an ongoing challenge that shapes the future of video compression, and I, as a researcher, am always eager to explore new frontiers in this evolving field.
More Posts from ai-videoupscale.com: