Upscale any video of any resolution to 4K with AI. (Get started for free)
VLC Streamer Bridging the Gap Between AI Video Upscaling and Cross-Platform Media Streaming in 2024
VLC Streamer Bridging the Gap Between AI Video Upscaling and Cross-Platform Media Streaming in 2024 - VLC 19 Integrates Nvidia RTX Video Super Resolution
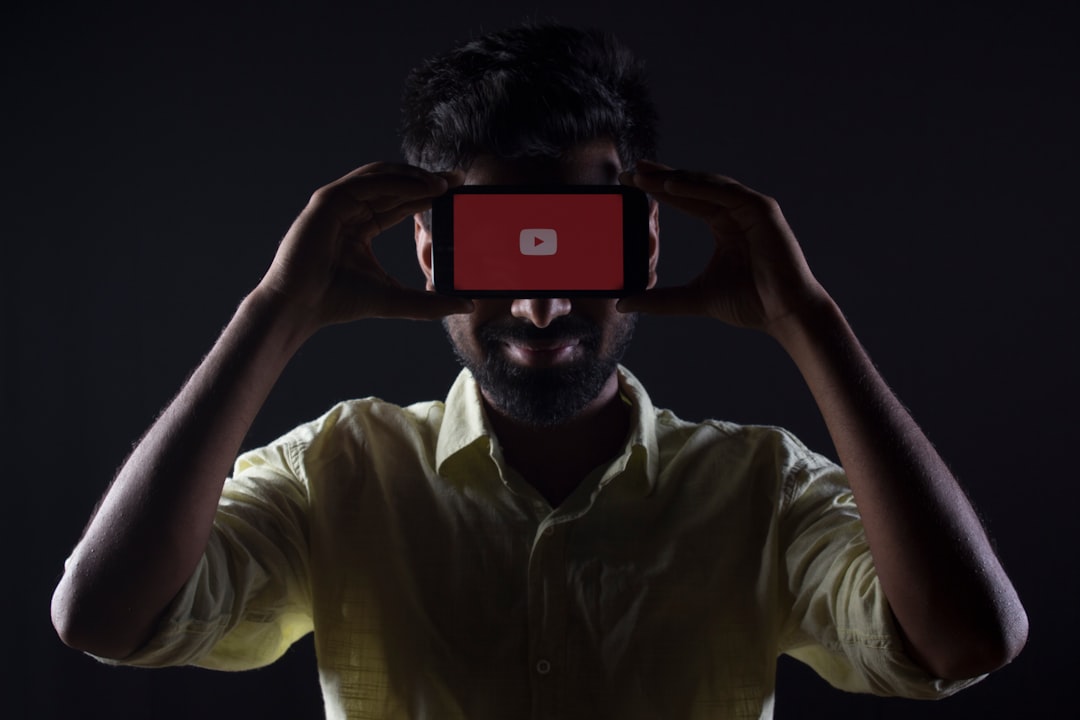
VLC Media Player version 19 now includes Nvidia's Video Super Resolution (VSR), a noteworthy step forward for video quality. This feature leverages the capabilities of Nvidia's RTX 30 and 40 series graphics cards to improve the resolution of lower quality videos. The AI-driven upscaling delivers sharper and more detailed visuals during playback. Users control this feature through the Nvidia Control Panel, providing a level of customization to their video viewing. Furthermore, this version of VLC has added HDR tonemapping, enhancing how high dynamic range content is displayed. It seems that AI-powered video enhancements are becoming more integrated into media players, bridging the divide between conventional playback and modern upscaling techniques. While this could improve viewing experiences, it's important to consider that this puts more strain on graphics hardware and may not be suitable for all users, especially those with less powerful systems. Whether or not these changes are a genuine improvement will depend on the quality of the source material, the capabilities of individual graphics cards, and user preferences.
VLC 19's inclusion of Nvidia's RTX Video Super Resolution (VSR) is an interesting development. It essentially uses AI to intelligently boost video quality by filling in missing details from lower-resolution sources. This approach goes beyond basic interpolation, using machine learning to reconstruct images with greater accuracy, a notable improvement over older techniques.
The feature's reliance on the horsepower of Nvidia's RTX 30 and 40 series GPUs is key to its functionality. These cards are optimized for real-time image manipulation, which is crucial for providing smooth, lag-free playback of upscaled video. It'll be interesting to see how well this approach handles high-action video, a traditional problem for upscaling algorithms.
One intriguing aspect is VLC's ability to leverage both open-source ideals and Nvidia's proprietary tech. This hybrid approach may set a pattern for future multimedia tools, showcasing how diverse technologies can collaborate. However, it is also worth noting the dependency this creates on specific hardware.
From an engineering standpoint, the adjustable settings within VLC for VSR are noteworthy. It allows users to fine-tune the quality/performance trade-off depending on their needs. This level of control could be useful in situations where you need to balance video quality with the limitations of certain systems.
The reliance on training the VSR technology with a wide range of content is a sign that it was designed to cope with the varied nature of video. This is also a potential advantage over older upscaling methods, though only time and testing will tell if this is a real advantage in practice. Also, the upcoming support for Nvidia's RTX Video HDR is intriguing as it suggests that further enhancements to visual quality through AI are in development.
It seems that VLC's RTX VSR integration addresses the current desire for high-quality video across a range of devices, offering a solution for enjoying higher-resolution content without relying on re-encoding or dedicated high-resolution sources. However, the overall impact and widespread adoption will depend on the actual effectiveness of the technology and how well it integrates into everyday workflows.
VLC Streamer Bridging the Gap Between AI Video Upscaling and Cross-Platform Media Streaming in 2024 - AI-Driven Upscaling Process Explained
AI-driven video upscaling has rapidly gained traction in 2024, becoming a popular method for improving video quality. This process relies on sophisticated machine learning algorithms to analyze low-resolution videos and reconstruct missing details, producing sharper and more refined images. It's a significant leap beyond basic interpolation methods, offering a more intelligent and nuanced approach to upscaling.
Several tools have emerged to implement this, catering to a range of needs. Some focus on readily available upscaling for everyday users, such as the 4K upscaling offered by CapCut and VideoProc Converter AI. Others, such as NVIDIA's RTX Video Super Resolution, are integrated into established media players like VLC, allowing users to leverage the power of their hardware to upscale videos during playback.
While these advancements are exciting, they also introduce a new set of considerations. The reliance on powerful GPUs can make this technology less accessible to users with less advanced systems. This raises questions about the balance between the visual benefits of AI upscaling and the potential strain it puts on computer resources. Ultimately, the effectiveness of AI-driven upscaling, and its overall impact on the future of video streaming, depends on the continued development of algorithms, improved accessibility, and a broader understanding of the trade-offs involved for various users.
AI-driven upscaling is increasingly being used to improve video quality, particularly for lower resolution content. It essentially works by using complex neural networks, like convolutional neural networks (CNNs), to analyze video frames and intelligently predict the missing pixel information needed to create a higher resolution output. The result is often a video that retains its original characteristics but appears much sharper and clearer.
One notable aspect of this process is its ability to reduce or eliminate artifacts common in low-resolution content. Things like blocky edges and blurring are often minimized through AI, leading to a more pleasing and natural appearance without causing obvious compromises in the video's integrity. However, how effectively this works can differ depending on the specific type of video. Animated videos, with their simpler visuals and distinct edges, tend to fare better than live-action footage, which often has a broader range of subtle details that are harder for the AI to reconstruct accurately.
The core of these AI models relies heavily on the vast amount of training data used to teach them. The more varied and diverse the content used during training, the better equipped the AI is to handle a wide range of video types. However, this creates a challenge when dealing with unique or niche video content that wasn't present in the training datasets. In such cases, AI upscaling may not yield the expected improvements, or it might introduce unforeseen issues.
Real-time AI upscaling has become possible thanks to advancements in graphics processing, particularly with GPUs. But this capability comes at a cost. Using AI for upscaling can require substantial computing power, potentially overwhelming older or less powerful systems and causing stuttering or lagging during playback. This factor may limit the accessibility of AI upscaling to those with newer and more capable computers.
Interestingly, many of these AI upscaling approaches are tuned towards a more human-centric notion of "quality". Instead of simply aiming for pixel-perfect reconstruction, which is computationally intensive, they prioritize results that look good to our eyes. This focus on "perceptual loss" during the training process prioritizes visual quality as perceived by humans rather than pure mathematical accuracy.
The rise of AI upscaling within software like VLC is quite significant, as it demonstrates the potential for open-source projects to keep pace with the cutting-edge technologies often found in proprietary software. It also suggests that the lines between open and closed platforms are blurring as technologies like AI become more accessible.
However, it's crucial to acknowledge that AI upscaling isn't without its potential drawbacks. It's possible that over-enthusiastic enhancement can sometimes lead to strange or undesirable results, such as a false sense of sharpness or the introduction of new forms of visual noise. This "over-sharpening" can make the video appear unnaturally detailed or even create new imperfections that weren't present in the original.
Another issue to consider is the increased processing demands which can introduce latency or lag during playback, potentially making the upscaled video less responsive. This latency can be a major concern for applications that require rapid responses, such as online gaming where smooth frame rates are critical to the overall experience.
Moving forward, the future of AI upscaling looks likely to incorporate more sophisticated feedback mechanisms. The algorithms powering these technologies could be refined over time based on the viewing experiences of individuals, leading to more personalized video experiences. This would mean that the upscaling process becomes increasingly adaptive to each user's preferences and viewing habits, allowing for a more finely tuned and optimal viewing experience.
VLC Streamer Bridging the Gap Between AI Video Upscaling and Cross-Platform Media Streaming in 2024 - Optimizing VLC for RTX VSR Performance
To get the most out of RTX VSR within VLC, you need to fine-tune settings both within VLC itself and in the NVIDIA Control Panel. Making sure the super resolution feature is active in the video image settings of the NVIDIA Control Panel unlocks the full potential of RTX 30 and 40 series graphics cards. The VSR quality slider, which goes from 1 (lightest) to 4 (most intense), lets you strike a balance between image quality and the load on your GPU, tailoring the experience to your system's capabilities. Additionally, setting VLC to be a high-performance application within the NVIDIA Control Panel can help make the video playback smoother, especially when using AI upscaling. It's important to recognize that leveraging these features often places higher demands on the system, so users should be mindful of their hardware limitations when exploring this advanced functionality.
VLC's recent integration of Nvidia's RTX Video Super Resolution (VSR) presents an intriguing opportunity to improve video quality using AI. This feature, powered by the Tensor Cores found in RTX 30 and 40 series GPUs, uses machine learning to upscale lower-resolution videos in real-time during playback. However, there's a trade-off between achieving the highest visual quality and maintaining smooth playback, especially on systems with less powerful hardware. Finding that sweet spot for performance and quality is a crucial aspect of optimizing VLC for RTX VSR.
One interesting aspect is how VLC's design allows for on-the-fly adjustments of VSR parameters. This lets users experiment with different levels of upscaling without needing to restart the video. It's quite useful for testing how various content types respond to upscaling. But it's important to remember that even with RTX VSR, the quality of the original video plays a crucial role. Videos that were already of poor quality might still have noticeable artifacts after upscaling, highlighting the inherent limits of the technology.
Furthermore, the algorithms that drive VSR are trained on a vast collection of videos. This means they're better at handling certain types of content over others. The source video's characteristics play a role in how effectively the upscaling happens. It's not always predictable how it will perform with obscure or niche video types. Also, the real-time feedback loop VLC uses while upscaling can lead to delays, especially during intense scenes. It's something to keep an eye on when assessing playback smoothness.
Enabling RTX VSR can significantly increase GPU energy consumption. It's important to be mindful of the potential for increased heat generation and power draw. Compatibility with various video codecs and compression types is another factor to consider. Heavier compression can sometimes hinder the upscaling process, leading to a less consistent improvement in visual fidelity.
The technology is constantly evolving. The future likely holds adaptive algorithms that are able to learn user preferences and tailor the upscaling process to specific hardware. However, this will require sophisticated user interfaces for practical implementation. In the meantime, testing the limits of RTX VSR with various types of media is essential. Exploring a wide range of content—from feature films and documentaries to cartoons and animated videos—can offer a better sense of the technology's strengths and weaknesses in diverse visual contexts.
By experimenting with different settings and content types, users can potentially refine their workflows and gain a better grasp of the potential benefits and limitations of this exciting technology. This approach is essential to understanding the real-world applications and implications of RTX VSR in everyday usage.
VLC Streamer Bridging the Gap Between AI Video Upscaling and Cross-Platform Media Streaming in 2024 - Anticipated RTX Video HDR Feature in VLC
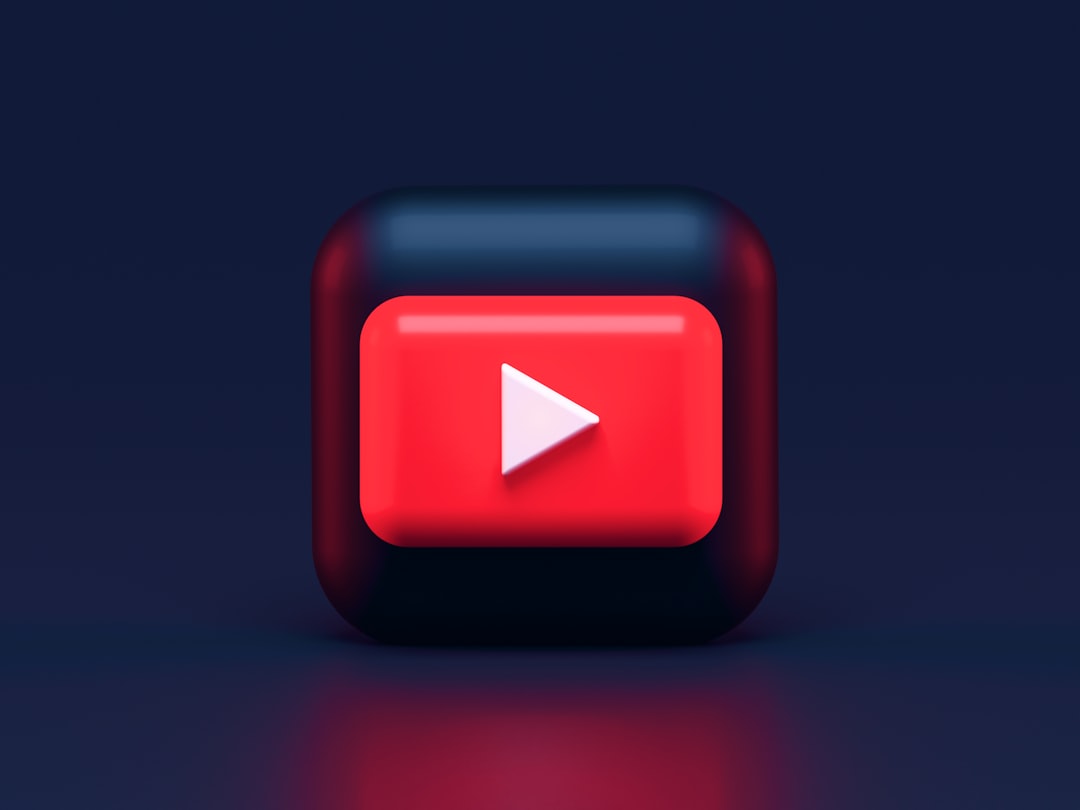
VLC Media Player is poised to integrate Nvidia's RTX Video HDR, a feature that's been anticipated by many. This addition promises a significant leap forward in video quality by using AI to transform standard dynamic range (SDR) video into HDR, creating a more immersive viewing experience. It's designed to work specifically with Nvidia's RTX 30 and 40 series graphics cards, fitting nicely alongside the existing VSR (Video Super Resolution) support within VLC. This pairing suggests a robust set of tools for refining video quality, though users should be mindful of the increased processing demands this technology places on their hardware. Older or less powerful systems may find that the benefits don't outweigh the strain it puts on their resources. While VLC continues to push the boundaries of video quality with these advancements, it's still crucial to consider both the source material and the capabilities of your hardware to truly understand the practical implications of these new AI-driven features.
VLC's upcoming RTX Video HDR feature, paired with its existing VSR support, seems to be a significant step in video quality enhancement. The idea of having HDR content not only upscaled but also tone-mapped within VLC is quite intriguing. This could lead to more visually rich experiences, especially when viewing HDR material on displays that don't inherently support it.
Nvidia's VSR relies on some clever neural network tricks that focus on reconstructing details rather than just filling in pixels with standard interpolation methods. This approach could lead to a more natural look in upscaled videos, though it remains to be seen how well it truly handles the diversity of video content.
While VSR aims to process video in real-time, its performance could be influenced by factors like video encoding and source quality. This is especially important to keep in mind for applications where responsiveness is key, like video editing or interactive streaming.
The combination of VSR and adaptive bitrate streaming is an interesting area to explore. Potentially, this technology could impact how bandwidth is managed during streaming. Maybe it'll lead to ways to optimize streaming services and reduce bandwidth usage when delivering lower resolution content.
VSR's reliance on Nvidia's tensor cores creates a tight coupling between hardware and software. This is a different approach to upscaling, one where the user experience is dynamically shaped by the available GPU resources. This raises questions about future developments in balancing performance and power consumption.
Despite VLC's focus on open-source principles, integrating Nvidia's proprietary technology can lead to potential compatibility challenges. This raises concerns about accessibility for users who don't use Nvidia hardware, which could create a fragmented experience across different platforms.
While VSR is aimed at minimizing artifacts, the type of source content will continue to play a large role in the final result. Highly compressed or noisy videos could still present challenges for the algorithm, potentially leading to new types of visual artifacts.
The ability to customize VSR within VLC gives users a lot of control, but it could also be confusing for those unfamiliar with technical settings. Balancing visual quality and performance effectively can be tricky and lead to inconsistent experiences for certain users.
The increased performance from VSR naturally results in more thermal output from GPUs. Understanding these thermal dynamics is particularly important for users with compact setups, as sustained high heat output could negatively impact system longevity.
The potential of the RTX VSR technology goes beyond video playback. It lays a groundwork that could be adapted to future technologies like VR and AR, or even be useful for enhancing gaming graphics in real-time, providing more visually immersive experiences. It's an interesting area to watch as the technology develops.
VLC Streamer Bridging the Gap Between AI Video Upscaling and Cross-Platform Media Streaming in 2024 - Cross-Platform Compatibility and User Experience
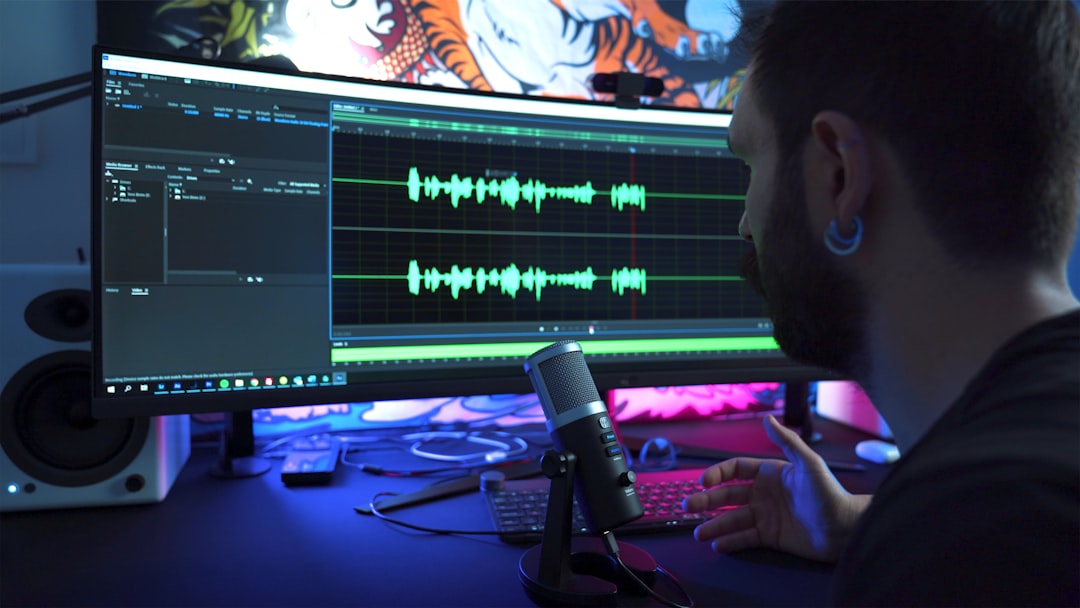
VLC's strength lies in its cross-platform compatibility, a feature that becomes even more important as features like Nvidia's RTX Video Super Resolution are incorporated. The updates to VLC enhance user experience across a variety of devices and operating systems, making it easy to play many different media formats without needing to install extra software. As VLC integrates AI-based video improvements, the way it's designed and the way the technology interacts with the user becomes incredibly important. But, the added features also place more demands on the hardware, potentially creating a less enjoyable experience for those with older devices. This is a departure from the historically accessible nature of VLC. Maintaining a smooth user experience regardless of system capabilities is critical for VLC's continued success as a core part of media streaming for a wide variety of users.
The goal of truly cross-platform compatibility in media streaming remains a challenge. While it's great to have software that works on various devices, often the experience isn't uniform. Devices with different hardware capabilities will naturally impact how smoothly a program like VLC operates. There's a noticeable difference in how smoothly a video might play on a high-end computer versus an older tablet or phone. This inconsistency in user experience across different platforms is a significant issue in the quest for ideal media consumption.
Research suggests a concerningly large number of users abandon apps due to issues with compatibility. It highlights how important it is for streaming services and applications to ensure their software functions as intended on a wide range of devices, not just the ones with the latest technology.
The push for cross-platform features sometimes seems to come at the cost of performance. AI-driven upscaling, though impressive, can put a strain on older or less powerful devices. The result can be choppy or slow playback, ruining what might otherwise be a great viewing experience.
How well users perceive a cross-platform experience is highly dependent on the application's design. It appears that interfaces need to adapt based on the type of device, like a phone or laptop. If a program's design isn't tailored to a specific device type, it can create a less-than-optimal user experience. This is especially important for streaming apps.
Upscaling technologies like VSR can produce variable results across devices. HDR features, for example, won't display properly on screens without sufficient display capabilities. The viewer might not experience what was intended by the content creator, which impacts how they perceive the content.
Higher resolutions usually come with higher data demands. This is particularly true for real-time applications like video games, where bandwidth and response times are essential. The consequence is potentially higher latency, making interactions in these types of applications slower or less smooth.
In today's world, media streaming is often reliant on subscription services. The ability to deliver content effectively across devices isn't just about enhancing the user experience; it's a major economic factor. If customers are dissatisfied with the experience, they may decide to cancel their subscriptions, which can have a significant financial impact.
There's a trend to use machine learning within these systems to adapt to users' specific habits and preferences. This creates a more personalized experience for users, but it also raises concerns about how this information is handled and the potential implications for privacy and security.
When a user streams video across different platforms, there are inevitable communication overheads. These overheads can be increased if different devices use varying data formats or protocols. This can lead to issues with latency and a decline in the quality of the media.
The specific codec used for compression and decompression can have a major impact on how effectively media streams across different platforms. Certain codecs might result in smaller file sizes, but if the devices aren't compatible with them, it can interfere with the ability to achieve a high visual quality during playback.
VLC Streamer Bridging the Gap Between AI Video Upscaling and Cross-Platform Media Streaming in 2024 - Future Developments in AI Video Processing for Streaming
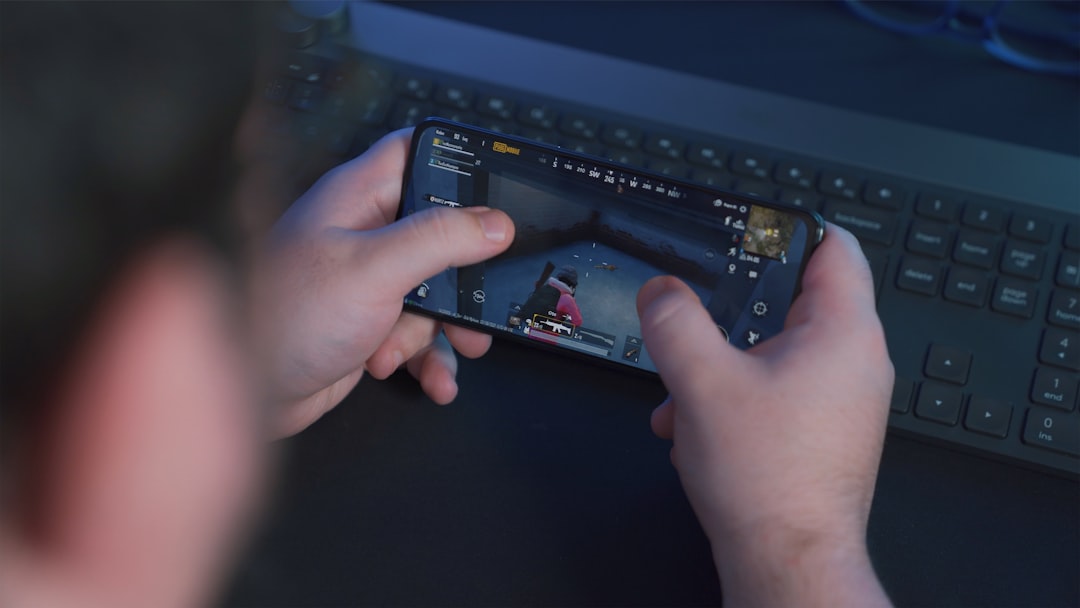
AI video processing for streaming is poised for significant advancements, particularly in personalized viewing experiences and real-time content adaptation. We can expect future developments to focus on refining algorithms to optimize video quality, delivering smoother and sharper video tailored to individual preferences. The integration of AI tools like NVIDIA's Maxine and features like VLC's RTX Video Super Resolution illustrate a growing trend of leveraging advanced hardware to achieve superior image quality and reduce latency in video streaming.
However, these improvements also bring about important considerations. Increased reliance on advanced hardware resources may create a divide between users with powerful setups and those with less capable devices, potentially impacting accessibility for a portion of users. This highlights the necessity for future AI-driven solutions to balance improvements in quality and performance with efficient resource management. The ultimate success of these developments hinges on developers' ability to strike a balance that ensures these advances are not only effective but also accessible and easy to use across a wide range of devices and systems. The challenge lies in creating AI-enhanced streaming experiences that are both impressive and practical for everyone, regardless of their hardware capabilities.
AI is fundamentally changing how we interact with video streaming, allowing for personalized experiences and dynamic content adjustments based on user engagement in real-time. This trend is extending into video production, where AI tools streamline workflows, reduce costs, and accelerate output, even potentially managing entire production pipelines independently. Platforms like NVIDIA's Maxine showcase the potential of cloud-based AI solutions to enhance video conferencing and streaming services. While the AI for video production market is anticipated to experience significant growth, reaching potentially billions of dollars by 2028, the integration of these tools into our everyday streaming experiences is still evolving.
The Real-Time Streaming Protocol (RTSP) remains vital in niche applications like surveillance and IP cameras due to its ability to deliver low-latency streaming. Yet, AI is now being incorporated into the broader video processing pipeline, impacting encoding, delivery, playback, and even monetization strategies within the streaming ecosystem. Techniques like frame interpolation and super-resolution, powered by AI, can help improve older video quality, particularly by addressing the limitations of lower frame rates. However, it's important to note that handling this vast flow of information at accelerated speeds comes with increased processing demands.
Looking ahead, we can expect AI video processing to continue adapting and enhancing the viewing experience through real-time content adjustments. These adjustments will likely be based on individual user interactions, further transforming how we interact with streaming platforms.
The complexity of the underlying neural networks, which are the backbone of many AI video upscaling techniques, is noteworthy. These networks are able to discern patterns in video that were previously difficult to identify with standard algorithms, which translates into improved detail recovery and better video quality after upscaling. Furthermore, many of the newer upscaling approaches incorporate not just the spatial aspects of a video, but also temporal information by referencing frames before and after the current one. This two-way approach creates more contextually accurate enhancements and improves motion coherence, reducing those distracting artifacts we often see with older techniques.
An interesting area of future development is the possibility of adapting the upscaling process based on each user's viewing habits and preferences. This personalized approach has the potential to fine-tune the experience, though it also raises questions about privacy and how this information is managed. There are also technical limitations. The computational demand of real-time AI upscaling can introduce some lag, particularly with fast-paced content like action sequences or sports. Striking a balance between quality and smooth playback is a challenge that continues to be addressed by researchers.
Another notable observation is that AI upscaling doesn't always deliver the same level of quality across different types of video content. In general, AI algorithms seem to do a better job upscaling animated video with its simpler visual elements compared to live-action, which often has a wider range of nuanced details that can be harder for the AI to accurately reconstruct. The quality of the source video is also a major factor, and the results of upscaling older, compressed videos are often less impressive. AI can do a lot, but it can't fully overcome the limits of low-quality source material. The added processing load from AI video features also increases the thermal load on systems. This is especially true for users who rely on compact systems or older hardware that may have inadequate cooling capabilities. Older and smaller systems may need to consider thermal management options to avoid performance issues or unexpected crashes.
The compression codec used for the original video also can have a big impact on upscaling. Certain formats might not be optimally suited for processing with AI, resulting in less-than-ideal upscaled outputs. With anticipated features like RTX Video HDR on the horizon, the future of video processing may incorporate more advanced techniques for managing color and contrast, further enriching the visual experience. However, this will likely bring additional demands for processing power and may not be compatible with all systems.
Finally, as new features become available, user interfaces will need to adapt to cater to a variety of skill levels. Streamlining the access to customization options without overwhelming users with technical jargon will be important for widespread adoption and general user satisfaction. The journey toward optimal AI-driven video processing will involve continuous development of algorithms, a focus on creating intuitive user interfaces, and a deeper understanding of the trade-offs involved for various users and systems.
Upscale any video of any resolution to 4K with AI. (Get started for free)
More Posts from ai-videoupscale.com: