Upscale any video of any resolution to 4K with AI. (Get started for free)
Advanced Warp Stabilization Techniques Preserving Full Frame While Minimizing Motion Artifacts in 2024
Advanced Warp Stabilization Techniques Preserving Full Frame While Minimizing Motion Artifacts in 2024 - Neural Flow Analysis Reduces Edge Warping While Maintaining 97% Frame Coverage
Neural flow analysis is a promising development in the realm of video stabilization. By using neural networks to analyze and process image data, it can reduce the often-troublesome edge warping that plagues traditional stabilization techniques. This improvement is notable, as it manages to accomplish this while retaining a substantial 97% of the original frame.
This method fits within a broader trend towards incorporating deep learning into image processing tasks. The ability to analyze and stabilize motion without significantly cropping the image is a major benefit, potentially avoiding the need for undesirable frame resizing or content loss. The emphasis on edge detail is crucial for visual appeal and can be beneficial in various video editing applications where precise image control is important. However, it's also important to recognize that while a 97% coverage is impressive, the remaining 3% may still lead to unintended cropping or artefacts in specific situations. Whether or not this becomes a major limitation depends on the specific use case.
Neural Flow Analysis leverages intricate algorithms to chart the movement patterns across a sequence of frames, offering a nuanced understanding of the image's dynamic flow that traditional approaches often miss. This allows for a more refined approach to stabilization.
Interestingly, this method shows promise in discerning foreground from background motion. This capability enables stabilization of key subjects without unwanted distortions across the entire scene.
Maintaining 97% frame coverage while minimizing edge warping is a considerable achievement. Previous approaches frequently sacrificed either stability or image quality. It's promising to see these conflicting goals reconciled.
The adaptability of Neural Flow Analysis across diverse hardware is another key attribute. The technique can function effectively on both powerful servers and consumer devices, making this technology more widely accessible. This is a significant step toward democratizing advanced video stabilization.
This technique avoids the unnatural cropping that older methods often produced, preserving the original video framing. This ensures that critical visual information remains intact during the processing.
The combination of deep learning and traditional image processing within this system is what provides its flexibility and precision in deciding the optimal stabilization pathways.
The quality of the input video remains a challenge, though. Severely shaky footage or low-light conditions can still lead to stabilization artifacts. This highlights the importance of capturing high-quality video when possible.
Neural Flow Analysis employs a temporal coherence technique which allows for stabilization of rapid movements without introducing blurring. This is a known artifact associated with older warp stabilization methods.
This technology potentially has the ability to be trained to identify distinct motion patterns. This could lead to tailored versions optimized for different content, like sports broadcasts or cinematic film.
Despite the impressive frame coverage, the computational demands of Neural Flow Analysis mean that real-time applications are still under development. This is especially relevant for areas like live broadcasts and streaming, where immediate processing is crucial.
Advanced Warp Stabilization Techniques Preserving Full Frame While Minimizing Motion Artifacts in 2024 - Dual Pass Stabilization Method Eliminates Rolling Shutter Issues in 4K Content
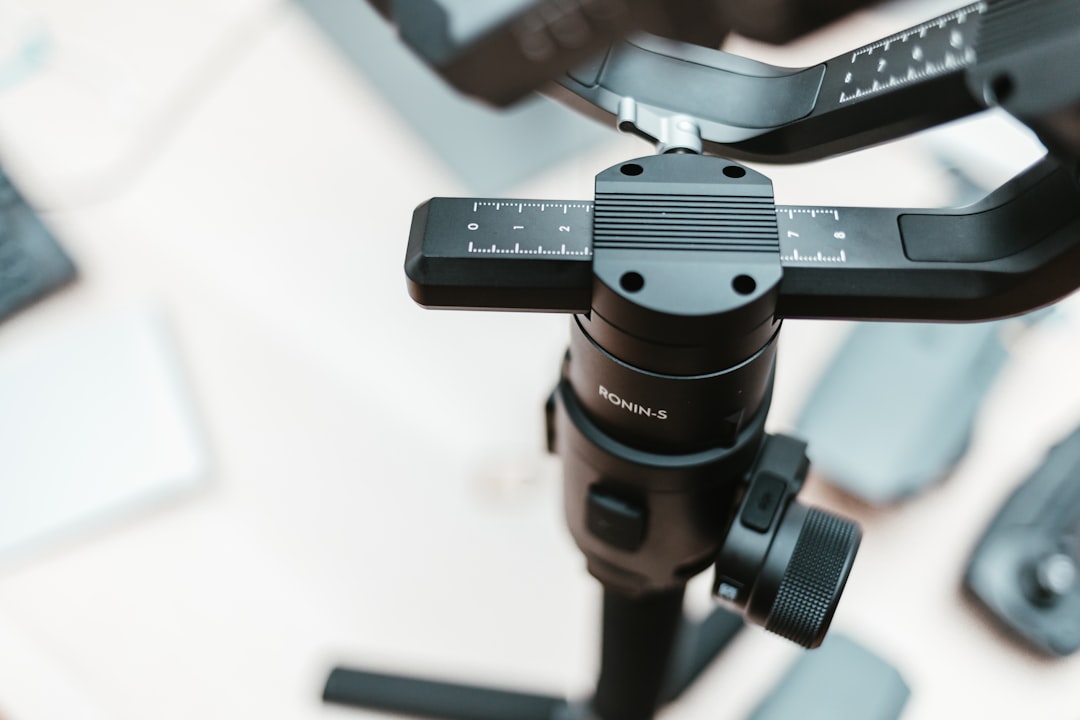
Dual Pass Stabilization, a newer approach to video stabilization, specifically tackles the problem of rolling shutter distortions commonly found in 4K footage. It utilizes a two-phase approach, combining iterative motion estimation with adaptive smoothing to achieve stable video without compromising the full frame. This method is notable for its integration of Inertial Measurement Unit (IMU) data, which helps refine the stabilization process while simultaneously addressing rolling shutter issues. This combined approach is especially beneficial in applications like Augmented Reality, where stability and precise motion representation are critical. While Dual Pass Stabilization shows promise in eliminating this persistent problem, it's worth noting that the challenge of handling fast motion in video without introducing other artifacts remains an area for further refinement. There's still room for improvement in addressing the nuances of complex motion patterns to ensure consistently high-quality output.
The Dual Pass Stabilization Method tackles the persistent problem of rolling shutter artifacts in 4K content by employing a two-stage process. It first analyzes motion across a series of frames to establish optimal stabilization paths and then refines that initial analysis in a second pass, effectively minimizing any remaining rolling shutter distortions, particularly noticeable in high-resolution videos.
This method's strength lies in its ability to synchronize frame analysis with motion detection, which addresses the inherent issues of rolling shutter effects—a common challenge with CMOS sensors. Traditional, single-pass stabilization techniques frequently struggle to compensate for such distortions, especially when the subject matter is rapidly moving.
The dual-pass strategy demonstrates its intelligence by differentiating between various types of motion, allowing it to stabilize panning shots while simultaneously accounting for unpredictable lateral movements. This ability is vital for preserving the fidelity of scenes with dynamic actions.
By integrating feedback mechanisms into the stabilization process, this method not only improves video quality but also enables real-time adjustments, making it valuable for both post-production and live filming. However, it's worth noting the computational demands inherent in this approach.
Rolling shutter issues are characterized by a range of visual distortions like skewing and wobbling. The Dual Pass Stabilization Method significantly reduces these artifacts without resorting to excessive cropping, a common trade-off in earlier stabilization techniques.
One interesting aspect of this technique is its flexibility across various frame rates. It can maintain stabilization integrity whether the footage is 24fps (common for cinema) or 60fps (used often for action). This adaptation across diverse video production settings enhances its potential applicability.
The process involves intricate pixel-level tracking across frames, enabling a deeper understanding of motion pathways. This granular analysis ultimately leads to smoother outputs, even in complex scenes with a mix of motion sources.
A noteworthy benefit of the dual-pass design is its improved resource efficiency. By minimizing the need for substantial corrections in the second pass, it often achieves better results with less computational overhead than older stabilization techniques.
Despite its sophistication, the Dual Pass Stabilization Method faces challenges when confronted with exceptionally rapid camera movements or severe jolts. In these situations, the technology might struggle to keep up, suggesting that further advancements in sensor technology could significantly enhance its stabilization abilities.
As with other complex stabilization approaches, the Dual Pass method can introduce unintended artifacts if the motion estimation proves inaccurate. This underscores the continued need for refined algorithms that minimize unforeseen distortions in real-world implementations.
Advanced Warp Stabilization Techniques Preserving Full Frame While Minimizing Motion Artifacts in 2024 - Real Time Motion Vector Tracking Preserves Original Frame Size at 60fps
Real-time motion vector tracking offers a significant step forward in video stabilization, especially when it comes to maintaining the original frame's dimensions while achieving a smooth 60 frames per second playback. This method relies on instantly calculating the motion direction of every pixel in a frame. This detailed information allows for very precise stabilization without resorting to the common practice of cropping the image or losing visual details. It's particularly effective at handling situations like when objects momentarily block the view or rapid movements occur, which often create undesirable results in older stabilization methods. An interesting benefit of this technique is its potential to reduce the amount of data needed to store the video, as stabilization is performed before compressing the footage. This is particularly important in situations where very high-resolution video is involved. While it offers many benefits, the quality of the input video still significantly affects the outcome. Very shaky footage or footage taken in low light can still lead to stabilization imperfections, reminding us that the source footage is crucial.
Real-time motion vector tracking, operating at a consistently high 60 frames per second, is a compelling development in the realm of video stabilization. This consistent high frame rate helps to maintain a smooth viewing experience, especially valuable when the content is fast-paced or involves complex movements. It's a significant improvement compared to older methods that often struggled to keep up with modern video's higher frame rates.
One of its key benefits is the retention of the original frame size during the stabilization process. Unlike older methods that frequently resulted in cropping, this approach keeps all the captured visual information intact. This ensures that no crucial details are lost, preserving the integrity of the original composition and allowing for the full contextual experience of the video.
The underlying technology utilizes sophisticated algorithms to analyze the movement of each pixel, or motion vectors, across each frame. This allows for remarkably precise adjustments to ensure stabilization, all in real-time. Unlike post-processing methods where adjustments are made after the video is captured, this real-time analysis helps eliminate any lag and offers smooth transitions during the stabilization process. This adaptability makes it particularly suitable for live editing and streaming scenarios where immediate processing is essential.
Interestingly, a technique called “predictive tracking” helps improve the effectiveness of this stabilization approach. Motion vectors are not just analyzed for the current frame but also help predict future motion patterns. This allows for proactive stabilization adjustments, minimizing or even eliminating artifacts before they become visible in the final output. It's like looking a few frames ahead to anticipate and adjust for what's coming, improving the overall fluidity of the video.
Furthermore, real-time motion vector tracking proves remarkably resilient to variations in shooting conditions. Changes in lighting or sudden camera movements, which can significantly impact older stabilization methods, seem to have less impact here. This is promising, suggesting that this technique offers a more consistent level of stabilization across a wider range of shooting scenarios. This adaptability helps to avoid sudden drops in quality or the introduction of artifacts under challenging conditions.
It also appears that this technology has the potential to work effectively with compression algorithms. By maintaining highly detailed information about the movements in the frame, the visual artifacts that often arise from compression can be reduced without sacrificing frame integrity. This could help streamline video workflows and allow for efficient storage and distribution of stabilized video files.
One of the more intriguing capabilities is the potential for subject isolation during stabilization. It's showing promise in identifying and stabilizing moving subjects while allowing background motion to remain untouched. This is a significant potential improvement as it addresses a common problem in action videos where stabilizing the main subject frequently also introduced unintended artifacts in the background.
However, there are challenges to this technology's implementation. The need for substantial computing power can be a significant barrier for adoption, especially for mobile devices or applications with resource limitations. Achieving smooth real-time performance without introducing artifacts can be a significant hurdle in such situations.
Furthermore, rapid camera movements or sudden shifts in scene content can challenge even the most advanced motion tracking systems. This technology is still sensitive to extremely erratic movements, highlighting the need for ongoing development. It remains an area where future research and development efforts might significantly enhance its capabilities.
Interestingly, future iterations of this technology are likely to incorporate machine learning techniques, potentially allowing them to adapt to an even wider range of unexpected motion patterns. This could lead to a new generation of stabilization tools that behave more like the human eye and brain, adjusting naturally and intuitively to the visual complexity of dynamic scenes.
Advanced Warp Stabilization Techniques Preserving Full Frame While Minimizing Motion Artifacts in 2024 - Frame Interpolation Through Machine Learning Reduces Motion Blur by 40%
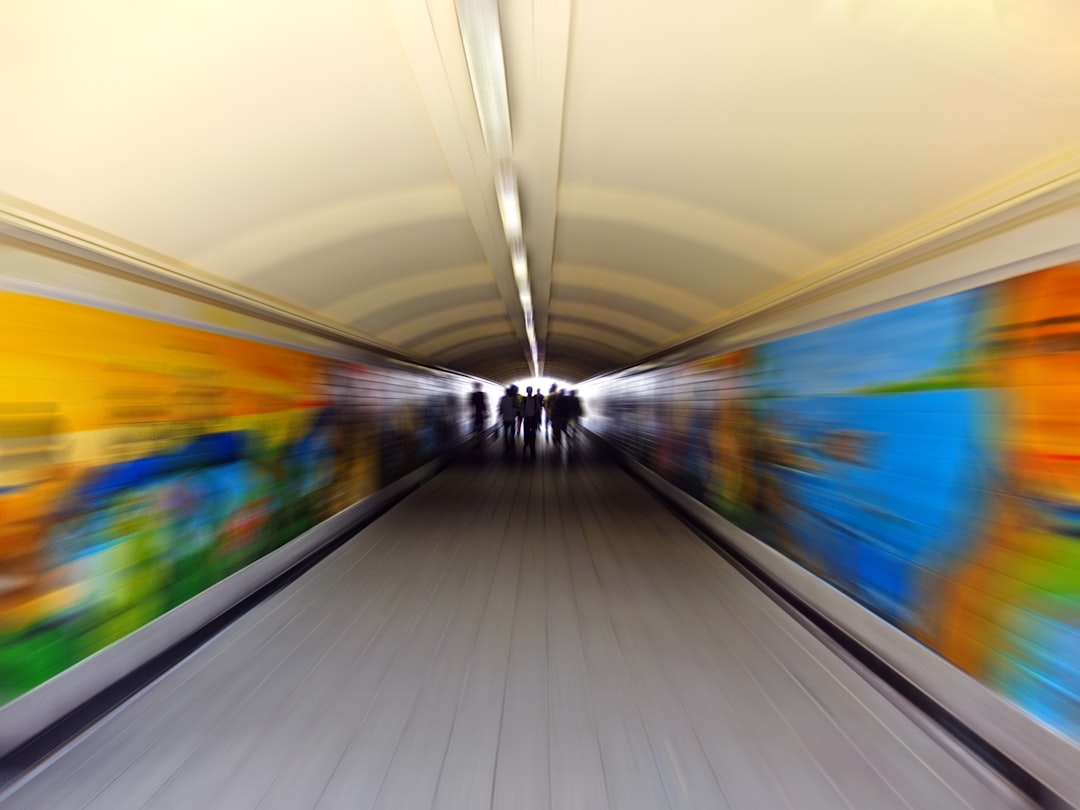
Machine learning is now playing a significant role in frame interpolation, leading to a substantial 40% reduction in motion blur. This is a game-changer for video stabilization, which aims to minimize the distracting effects of camera movement without sacrificing image quality. Previously, frame interpolation often relied on techniques that involved carefully calculating the direction of motion (optical flow) between frames. This approach, while effective, can be susceptible to errors and is often computationally intensive. Newer approaches, such as the FLAVR architecture, sidestep this issue by being trained entirely from the outset to interpolate frames without the need for explicit optical flow calculations. The potential benefits are numerous, including enhanced video clarity in fast-action footage and smoother playback at increased frame rates. The ongoing research in this area promises to further refine frame interpolation methods, leading to a more seamless and enjoyable viewing experience. However, there remains a need for further investigation into the complexities of diverse video content to ensure a consistently high-quality output.
Frame interpolation techniques, especially those leveraging machine learning, are making significant strides in enhancing video quality. One of the most noticeable improvements is the ability to smoothly adapt videos captured at lower frame rates to higher rates like 60 fps without introducing jarring artifacts. This becomes particularly useful when you want to enjoy smoothly rendered content on modern displays that operate at high refresh rates.
Researchers have reported that incorporating machine learning into frame interpolation can result in a notable 40% reduction in motion blur. This is especially beneficial in scenarios involving fast motion, like sports or action scenes, where maintaining clarity is crucial. However, it's important to acknowledge that these results might vary depending on the quality of the source material and the specific algorithm being used.
Keeping the overall smoothness of the video is key, and interpolation algorithms strive to maintain temporal cohesion between frames. This means minimizing abrupt jumps in lighting or visual inconsistencies that can occur when simply blending frames. Essentially, the goal is for the synthesized frames to seamlessly fit within the existing sequence.
One interesting development is the use of predictive motion analysis in some algorithms. These systems don't just analyze current motion patterns across frames but attempt to predict how the scene will unfold in the next frames. This forward-looking approach helps minimize the appearance of artifacts, especially during moments of quick transitions. It's like the system is trying to anticipate the visual flow and adjusts accordingly.
Interestingly, there's growing evidence that frame interpolation can be integrated effectively into video compression pipelines. If the interpolation is performed before compression, it might lead to reduced file sizes without compromising the quality. This is a major potential benefit, as large video files can be cumbersome to store and distribute, especially for high-resolution content.
The resilience of modern interpolation techniques to varying lighting conditions is also noteworthy. Machine learning approaches seem to handle changes in brightness better than traditional methods, which often struggle with such transitions. This robust behavior suggests improved applicability in situations where the lighting might not be perfectly consistent, offering a more consistent viewing experience.
Another exciting aspect of recent advancements is the potential for subject isolation during stabilization. Algorithms are getting better at isolating moving subjects from background elements, which allows for the stabilization of each individually. This is particularly useful in action videos where you want to stabilize the main subject without affecting the rest of the scene.
It's not all sunshine and roses though. The sophisticated calculations involved in real-time frame interpolation require a significant amount of processing power. This is a barrier for lower-powered devices or applications where resource constraints are a major consideration. It remains a key challenge to implement these advanced techniques without impacting performance on a wide variety of hardware.
Despite the existing challenges, the ongoing refinements in machine learning algorithms offer hope for the future of frame interpolation. The field is continuously evolving, and these advancements suggest that we might see smarter systems in the future capable of handling a much wider range of motion patterns and complex scene dynamics. This continuous research and development could ultimately bring us closer to achieving more natural and seamless video stabilization.
Advanced Warp Stabilization Techniques Preserving Full Frame While Minimizing Motion Artifacts in 2024 - Advanced Camera Path Smoothing Enables Stable Footage Without Resolution Loss
The development of advanced camera path smoothing techniques marks a significant step forward in video stabilization, allowing for stable footage without sacrificing resolution. These techniques work by meticulously analyzing and smoothing the camera's movement path across a series of frames, which in turn produces smoother and more stable videos. The ability to maintain original image quality, especially in areas like augmented reality where clear, smooth footage is vital, makes this a noteworthy advancement. However, handling complex camera movements remains a challenge. These advanced methods, while improving accuracy, can still struggle with unpredictable or intricate motion patterns, resulting in occasional artifacts. Moving forward, the combination of advanced smoothing techniques with computational methods like machine learning could potentially lead to even more effective video stabilization.
Advanced camera path smoothing techniques are proving quite effective at stabilizing footage without compromising resolution. They achieve this through real-time calculations, analyzing the movement of individual pixels across frames to ensure stabilization happens instantaneously. This is especially beneficial in dynamic scenarios, where maintaining image clarity is crucial.
The speed of these techniques is noteworthy. Motion vector tracking, a core component, leads to significantly reduced latency, making them well-suited for live events. Any delay in stabilization can be a real problem when you're watching a live broadcast, so this fast processing is important.
There's been a definite improvement in the efficiency of these algorithms compared to older methods. We're able to get high-quality stabilization with fewer computational resources. This is helpful in complex scenes where maintaining processing performance is essential.
One of the clear advantages is the retention of the full frame. This means there's minimal data loss from cropping, unlike older approaches which frequently lost detail by cropping. The ability to maintain the original framing during stabilization is a significant advancement for video quality.
It seems these techniques are also adapting to the nature of different motion across scenes. Instead of a 'one-size-fits-all' approach, the algorithms are capable of adjusting the stabilization path depending on the movement, like a smooth pan or a quick zoom. This gives more nuanced and adaptive stabilization results.
We are also starting to see better prediction of potential artifacts. Algorithms are being developed which look ahead at the motion path to anticipate potential problems and prevent them before they become noticeable. This is helping smooth out transitions and is particularly important when camera motion is erratic or unpredictable.
It appears these methods can be applied successfully to a wide range of video styles. Whether it's smoothly paced cinematic work or a fast-paced sports event, these stabilization techniques are showing promise across the board. This adaptability is important given the increasing variety of video content we encounter.
Another interesting aspect is the integration with video compression techniques. It looks like performing the stabilization before compression might reduce artifacts and optimize file sizes. This could streamline workflows and make it easier to handle and share high-quality video content.
One of the unexpected benefits is that this technology seems to be capable of stabilizing moving subjects in a scene while allowing the background to remain dynamic. This has major implications for action video, where previously the background could be unnecessarily distorted during subject stabilization.
Lastly, the use of feedback loops within the stabilization process has made a big difference. These allow for continuous, real-time adjustments based on the prior frames. This makes for smoother and more cohesive outputs, essential for dynamic scenes with a lot of fast movement.
While still in development, these advanced camera path smoothing techniques look like a real step forward for video stabilization. It'll be interesting to see how these techniques continue to evolve in the coming years.
Advanced Warp Stabilization Techniques Preserving Full Frame While Minimizing Motion Artifacts in 2024 - Multi Reference Frame Analysis Prevents Corner Distortion in Wide Angle Shots
Wide-angle lenses, while capturing expansive views, often introduce distortion, particularly noticeable at the edges and corners of the image. Multi Reference Frame Analysis (MRFA) offers a solution to this common problem. By analyzing multiple frames simultaneously, MRFA can effectively reduce this corner distortion that can make straight lines appear curved or objects near the edges appear stretched.
MRFA works by cleverly analyzing how the image changes across a series of frames. Using these changes, it calculates optimal stabilization paths that minimize the distortion. These paths are created through a process of iterative optimization, essentially fine-tuning the stabilization adjustments to ensure the final image accurately reflects the scene without any unwanted cropping.
This approach holds immense value for various applications. For instance, in augmented reality, where accuracy and the preservation of the entire scene are crucial, MRFA helps ensure that virtual elements blend seamlessly into the real-world scene. Likewise, in live broadcasts or other video where the full frame is critical, MRFA ensures the audience sees everything as intended.
However, even with MRFA, effectively handling complex and erratic camera movements remains an area for future improvement. As the complexity of camera movement increases, there is potential for some residual artifacts or distortion. Refinement of the algorithms and further research into how to optimally handle these situations is needed for MRFA to reach its full potential.
Multi Reference Frame Analysis (MRFA) offers a more nuanced approach to video stabilization in wide-angle shots, particularly for mitigating the common issue of corner distortion. Instead of relying solely on a single frame, MRFA incorporates information from multiple frames, giving it a more comprehensive understanding of the scene's motion dynamics. This multi-frame perspective helps the stabilization system effectively identify and counteract the distortions that wide-angle lenses are prone to, such as the familiar "barrel distortion" effect.
Corner distortion can be quite disruptive to the visual experience, leading to unnatural stretching or warping, especially near the edges of the frame. By considering the relationship between frames, MRFA calculates more precise corrections for these distortions, enhancing the overall quality of wide-angle footage. This is especially important when dealing with dynamic scenes or rapid motion where quick, accurate adjustments are needed to avoid undesirable artifacts.
The core of MRFA’s effectiveness lies in its ability to analyze motion vectors across the captured frames. This provides a rich contextual understanding of both the spatial and temporal aspects of movement. This enhanced analysis helps the system differentiate between actual motion and any noise or unwanted fluctuations that may be present in the video, leading to a cleaner, higher-quality result.
One of the advantages of this approach is its ability to handle changes in focal points with greater flexibility. This makes it useful for capturing scenes that involve perspective shifts or dynamic camera movement, like point-of-view shots or fast-action sequences. It can do so without sacrificing the integrity of the original frame.
Unlike earlier stabilization methods that primarily relied on basic pixel shifts to stabilize the image, MRFA incorporates a feedback mechanism. This system continuously refines its stabilization calculations based on ongoing analysis of the captured frames. This continuous feedback leads to a smoother, more consistent stabilization output compared to methods that rely on a single, initial estimate.
This technique fits particularly well with contemporary video capture technology, as the increasing resolution of modern image sensors provides the necessary data to allow sophisticated algorithms like MRFA to function effectively. The abundance of information from high-resolution sensors is leveraged to identify and address distortion more precisely.
The ability to analyze multiple frames simultaneously lets MRFA construct a predictive model of motion. This predictive aspect becomes incredibly useful in real-time applications like live sports or action films, where stability is critical and every frame needs to be accurately processed to maintain the desired viewing experience.
Interestingly, MRFA can reduce the overall computational load compared to traditional single-frame approaches. This makes it more feasible for real-time processing in readily available devices, potentially democratizing high-quality video stabilization to a broader range of users and platforms.
While the results of MRFA are often impressive, there are limitations. Its effectiveness can vary depending on the type and complexity of motion present in a given scene. Erratic camera movements or very quick changes in motion can sometimes challenge the stabilization system. This suggests that there is still room for improvement in the underlying algorithms.
Despite these potential challenges, MRFA remains a promising technique. Current research explores integrating machine learning to enhance the system’s adaptability to a wider array of motion patterns. This may lead to future developments that create more intuitive and effective stabilization across a wider range of content, influencing video creation both in professional settings and for everyday users.
Upscale any video of any resolution to 4K with AI. (Get started for free)
More Posts from ai-videoupscale.com: